Scientific and technical journal
«Oilfield engineering»
ISSN 0207-2351
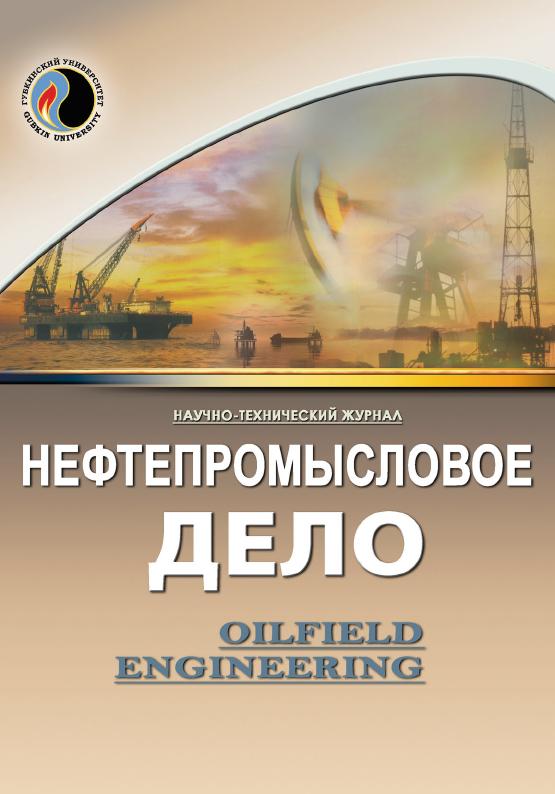
Dynamic analysis of oil fields development based on artificial neural network of error back propagation
UDC: 550.8.052
DOI: 10.33285/0207-2351-2023-5(653)-13-20
Authors:

1 National University of Oil and Gas "Gubkin University", Moscow, Russia
2 Institute of Oil and Gas Problems of the Russian Academy of Sciences
Keywords: production dynamics, dynamic models, database analysis, neural network, method of an error back propagation
Annotation:
Currently, the deterioration of hydrocarbon reserves structure is observed in the world. Most of the unique and large fields located in the oil regions of the world are in the late and final stages of development. After primary and secondary oil production, a significant amount of oil remains in the reservoir rocks in the form of residual oil. Under such conditions, an accurate forecast of oil production is required to increase the economic efficiency of oil fields operation, to ensure rational and scientifically-based development management. To solve this problem, the article proposes a new method for dynamic oil recovery analysis based on the neural network of an error back propagation using logging results, production history, etc. to create a database. The neural network modeling technology involves the creation of a predictive model based on all field data. Principal component analysis allowed the selection of the most representative indicators as input factors. The oil flow rate was taken as the predicted value. The forecast is carried out using a neural network of an error back propagation. It is shown that the maximum error of oil production forecast is less than 8,5 %. The forecast demonstrates a good result, has practical and scientific significance.
Bibliography:
1. Application of Artificial Intelligence Techniques in Drilling System Design and Operations: A State of the Art Review and Future Research Pathways / O. Bello, C. Teodoriu, T. Yaqoob [et al.] // Paper presented at the SPE Nigeria Annual International Conference and Exhibition, Lagos, Nigeria, August 2016. – URL: doi: https://doi.org/10.2118/184320-MS
2. A Novel Adaptive Non-Linear Regression Method to Predict Shale Oil Well Performance Based on Well Completions and Fracturing Data / A. Bakshi, E. Uniacke, M. Korjani, I. Ershaghi // Paper presented at the SPE Western Regional Meeting, Bakersfield, California, April 2017. – URL: https://doi.org/10.2118/185695-MS
3. Noshi C., Schubert J. The Role of Machine Learning in Drilling Operations; A Review // Paper presented at the SPE/AAPG Eastern Regional Meeting, Pittsburgh, Pennsylvania, USA, October 2018. – URL: https://doi.org/10.2118/191823-18ERM-MS
4. Causal Analysis and Data Mining of Well Stimulation Data Using Classification and Regression Tree with Enhancements / S. Bhattacharya, M. Maucec, J. Yarus [et al.] // Paper presented at the SPE Annual Technical Conference and Exhibition, New Orleans, Louisiana, USA, September 2013. – URL: https://doi.org/10.2118/166472-MS
5. Data Analytics for Production Optimization in Unconventional Reservoirs / M. Srikanta, J. Schuetter, Zhong Ming, R. LaFollette // Paper presented at the SPE/AAPG/SEG Unconventional Resources Technology Conf., San Antonio, Texas, USA, July 2015. – URL: https://doi.org/10.15530/URTEC-2015-2167005
6. Gupta S., Fuehrer F., Jeyachandra B.Ch. Production Forecasting in Unconventional Resources using Data Mining and Time Series Analysis // Paper presented at the SPE/CSUR Unconventional Resources Conference, Calgary, Alberta, Canada, September 2014. – URL: https://doi.org/10.2118/171588-MS
7. Gafarov F.M., Galimyanov A.F. Iskusstvennye neyronnye seti i prilozheniya: ucheb. posobie. – Kazan’: Izd-vo Kazan. gos. un-ta, 2018. – 121 s.
8. Shuvalov N.V., Khrustalev A.O., Zamuruev I.N. Neyronnye seti // Alleya nauki. – 2017. – № 5. – S. 159–165.
9. Tablichnaya realizatsiya iskusstvennoy neyronnoy seti radial’nykh bazisnykh funktsiy dlya klassifikatsii obraztsov / O.V. Anikina, O.M. Gushchina, E.V. Panyukova, N.N. Rogova // Sovremennye informatsionnye tekhnologii i IT-obrazovanie. – 2018. – T. 14, № 2. – S. 436–445. – DOI: 10.25559/SITITO.14.201802.436-445
10. Study on Prediction Model of Grain Yield Based on Principal Component Analysis and BP Neural Network / Xu Xing Mei [et al.] // Applied Mechanics and Materials. – 2015. – Vol. 713–715. – Pp. 1939–1942. – doi:10.4028/www.scientific.net/amm.713-715.1939
11. Ivanova M.A. Realizatsiya postroeniya razbieniya Voronogo dlya OSRV MAKS. – SPb., 2018. –25 s.
12. Kolesov V.V., Kurganov D.V. Raschet reytinga skvazhin-kandidatov pri uplotnyayushchem burenii s pomoshch’yu mashinnogo obucheniya na promyslovykh dannykh (metod opornykh vektorov) // Tekhnicheskie nauki. – 2019. – № 1(61). – S. 7–19.
13. Karabtsev S.N., Stukolov S.V. Postroenie diagrammy Voronogo i opredelenie granits oblasti v metode estestvennykh sosedey // Vychislitel’nye tekhnologii. – 2008. – T. 13, № 3. – S. 65–80.
14. Analiz zavisimosti rezul’tatov matematicheskogo modelirovaniya razrabotki neftyanykh mestorozhdeniy ot iskhodnykh dannykh na primere modeli dvukhfaznoy fil’tratsii / N.P. Efimova, I.V. Afanaskin, A.A. Kolevatov [i dr.] // Vestnik kibernetiki. – 2018. – № 3(31). – S. 58–67.