Scientific and technical journal
«Automation and Informatization of the fuel and energy complex»
ISSN 0132-2222
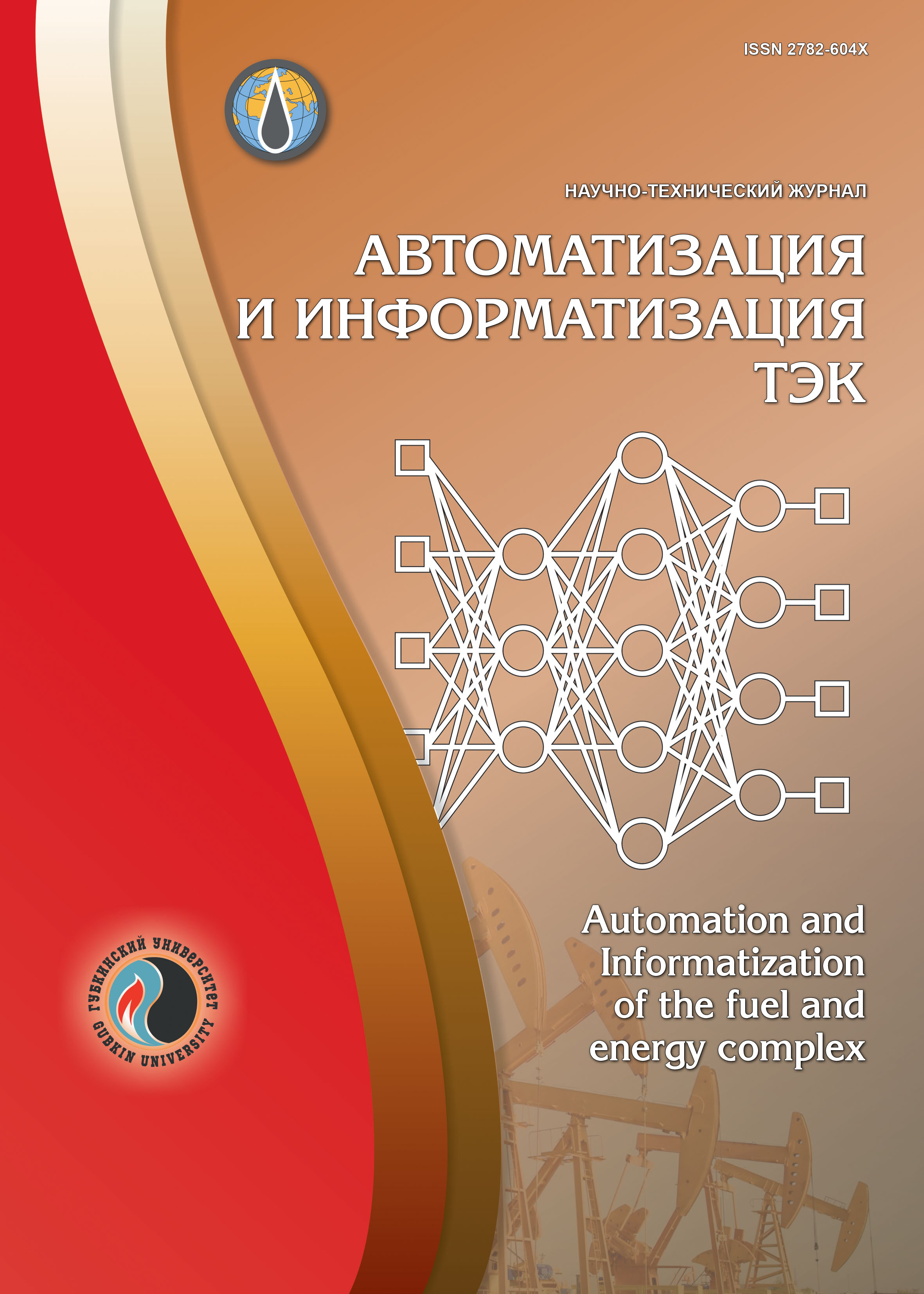
On the methodology of application of neural networks mathematical models when solving problems of the oil and gas complex
UDC: 519.673:622.691.4
DOI: 10.33285/2782-604Х-2022-2(583)-28-35
Authors:


1 National University of Oil and Gas "Gubkin University", Moscow, Russian Federation
Keywords: neural network algorithms, mathematical modeling, flows in reservoirs, gas transportation systems, simulators
Annotation:
The apparatus of neural networks is being intensively developed and successfully applied to solving practical problems of the oil and gas complex. Neural network algorithms are widely used in the field of complexly formalized, weakly formalized and non-formalized problems. Numerous successful examples of neural network algorithms lead to the illusory impression that the success of any of their applications is guaranteed a priori. The article is aimed at dispelling of this misconception and, as far as possible, preventing attempts to build neural network algorithms, which are doomed to failure in advance, where this is not justified by the technological essence of the problem. Some examples are given, two series of publications are analyzed, one of which relates to the problem of developing oil and gas fields, and the other one – to the operational control of gas transmission pipeline systems. It is proved that the neural network algorithms proposed in the publications cannot replace and, moreover, improve the existing methods for solving production problems.
Bibliography:
1. Galushkin A.I. Neyronnye seti: osnovy teorii. – M.: Goryachaya liniya-Telekom, 2010. – 496 s.2. Khaykin S. Neyronnye seti: polnyy kurs = Neural Networks: A Comprehensive Foundation. – 2-e izd. – M.: Vil'yams, 2006. – 1104 s.
3. Aramesh A., Montazerin N., Ahmadi A. A general neural and fuzzy-neural algorithm for natural gas flow prediction in city gate stations // Energy and Buildings. – 2014. – Vol. 72. – P. 73–79. – DOI: 10.1016/j.enbuild.2013.12.020
4. Artificial neural network models for predicting condition of offshore oil and gas pipelines / M.S. El-Abbasy, A. Senouci, T. Zayed [et al.] // Automation in Construction. – 2014. – Vol. 45. – P. 50–65. – DOI: 10.1016/j.autcon.2014.05.003
5. Base Oil Process Modelling Using Machine Learning / M.A.M. Fadzil, H. Zabiri, A.A. Razali [et al.] // Energies. – 2021. – Vol. 14, Issue 20. – DOI: 10.3390/en14206527
6. Somu N., Raman G., Ramamritham K. A hybrid model for building energy consumption forecasting using long short term memory network // Applied Energy. – 2020. – Vol. 261. – DOI: 10.1016/j.apenergy.2019.114131
7. Modeling and forecasting building energy consumption: A review of datadriven techniques / M. Bourdeau, X. Zhai, E. Nefzaoui [et al.] // Sustainable Cities and Society. – 2019. – Vol. 48. – DOI: 10.1016/j.scs.2019.101533
8. Anđelković A.S., Bajatović D. Integration of weather forecast and artificial intelligence for a short-term city-scale natural gas consumption prediction // J. of Cleaner Production. – 2020. – Vol. 266. – DOI: 10.1016/j.jclepro.2020.122096
9. Prado F., Minutolo M.C., Kristjanpoller W. Forecasting Based on an Ensemble Autoregressive Moving Average – Adaptive Neuro – Fuzzy Inference System – Neural Network – Genetic Algorithm Framework // Energy. – 2020. – Vol. 197. – DOI: 10.1016/j.energy.2020.117159
10. Qian D., Fox P.H., See B.L. Accurate Natural Gas Load Hourly Forecasting Using ANN Model Trained with Multiple Parameters. – URL: https://search.spe.org/i2kweb/SPE/doc/onepetro:15E05D32/
11. Umanovskiy A.V. Sostyazatel'nye svertochnye neyronnye seti v kachestve evristicheskoy modeli protsessa dvukhfaznoy fil'tratsii v poristoy srede // Vychislitel'naya mekhanika sploshnykh sred. – 2020. – № 2(13). – S. 231–241. – DOI: 10.7242/1999-6691/2020.13.2.18
12. Umanovskiy A.V. Metodika imitatsionnogo modelirovaniya na osnove obuchayushchikh dannykh dlya dvukhfaznogo techeniya v geterogennoy poristoy srede // Komp'yuternye issledovaniya i modelirovanie. – 2021. – T. 13, № 4. – S. 779–792. – DOI: 10.20537/2076-7633-2021-13-4-779-792
13. Umanovskiy A.V. Perspektivy primeneniya sistem iskusstvennogo intellekta v zadachakh modelirovaniya neftegazovykh mestorozhdeniy // Filosofiya iskusstvennogo intellekta – 2018: nauch. tr. Mezhdunar. molodezhnoy mezhdistsiplinarnoy konf., Moskva, 12-13 apr. – 2018.
14. Umanovskiy A.V. Glubokie neyronnye seti dlya uskoreniya fizicheskoy simulyatsii flyuidov v poristoy srede // IV region. nauch.-tekhn. konf. "Gubkinskiy universitet v reshenii voprosov neftegazovoy otrasli Rossii", posvyashchennoy 90-letiyu Gubkinskogo un-ta i fakul'teta ekonomiki i upravleniya: sb. tez. dokl., 22-23 okt. – M.: Izd. tsentr RGU nefti i gaza (NIU) im. I.M. Gubkina, 2020. – S. 292.
15. Kratkosrochnoe stokhasticheskoe prognozirovanie dinamiki potokov i zapasov gaza v Edinoy sisteme gazosnabzheniya Rossii na osnove rekurrentnykh neyronnykh setey / N.A. Kislenko, A.V. Belinskiy, A.S. Kazak, O.I. Belinskaya // Avtomatizatsiya, telemekhanizatsiya i svyaz' v neftyanoy promyshlennosti. – 2021. – № 9(578). – S. 27–35. – DOI: 10.33285/0132-2222-2021-9(578)-27-35
16. Kislenko N.A., Belinskiy A.V., Kazak A.S. Metody, algoritmy i instrumenty modelirovaniya i optimizatsii rezhimov raboty Edinoy sistemy gazosnabzheniya Rossii na osnove tekhnologiy iskusstvennogo intellekta. Chast' 1 // Gazovaya prom-st'. – 2021. – № 9(821). – S. 88–96.
17. Kislenko N.A., Belinskiy A.V., Kazak A.S. Metody, algoritmy i instrumenty modelirovaniya i optimizatsii rezhimov raboty Edinoy sistemy gazosnabzheniya Rossii na osnove tekhnologiy iskusstvennogo intellekta. Chast' 2 // Gazovaya prom-st'. – 2021. – № 10(822). – S. 98–104.
18. Sukharev M.G., Stavrovskiy E.R. Raschety sistem transporta gaza s pomoshch'yu vychislitel'nykh mashin. – M.: Nedra, 1971. – 205 s.
19. Sukharev M.G., Stavrovskiy E.R. Optimizatsiya sistem transporta gaza. – M.: Nedra, 1975. – 277 s.
20. Sukharev M.G., Popov R.V., Bal'chenko A.S. Problemy mnogokriterial'nogo upravleniya mnogonitochnymi koridorami gazoprovodov // Matematicheskie modeli i metody analiza i optimal'nogo sinteza razvivayushchikhsya truboprovodnykh i gidravlicheskikh sistem: tr. XV Vseros. nauch. seminara, 5–11 sent. – Irkutsk: ISEM SO RAN. – 2016. – S. 283–304.
21. Sukharev M., Popov R., Balchenko A. Multiple-criteria decision analysis for cross-country gas pipelines // E3S Web of Conferences. – 2019. – Vol. 102. – DOI: 10.1051/e3sconf/201910203010
22. Makarov A.A. Achievements and Challenges of Systems Studies on Energy Development in Russia, Their Possibilities in the "Digital" Society // Energy Systems Research. – 2021. – Vol. 4, No. 3. – P. 79–83. – DOI: 10.38028/esr.2021.03.0008