Scientific and technical journal
«Automation and Informatization of the fuel and energy complex»
ISSN 0132-2222
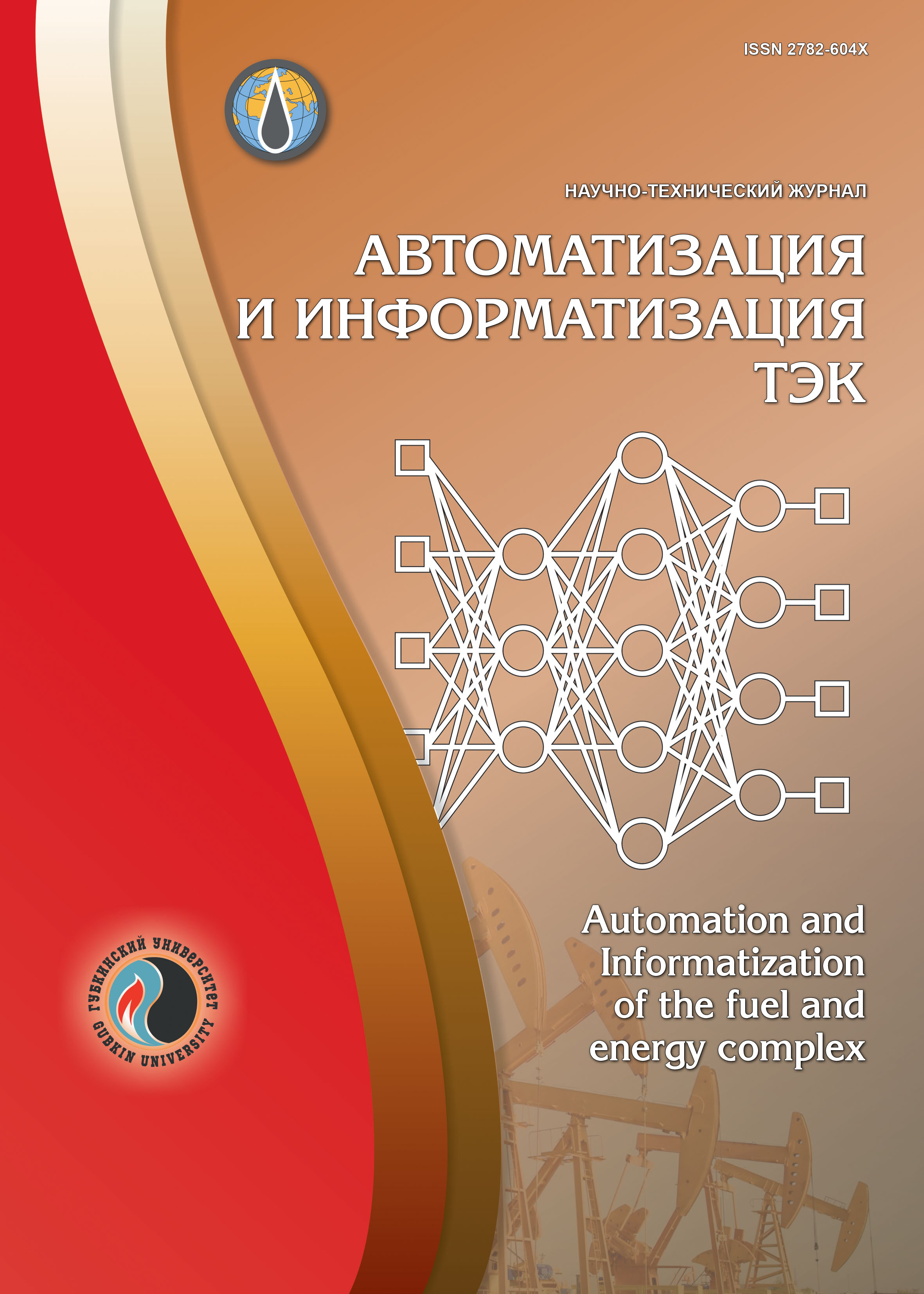
The validity of the application, the current state and some prospects for the development of neural network models of the Unified Gas Supply System of Russia
UDC: 519.673
DOI: 10.33285/2782-604X-2022-5(586)-6-17
Authors:



1 Gazprom", St. Petersburg, Russian Federation
2 NIIgazekonomika, Moscow, Russian Federation
Keywords: gas transmission system (GTS), Unified Gas Supply System (UGSS), mathematical modeling, optimization, artificial intelligence, machine training, neural networks
Annotation:
The progress in the development of modern computer technologies opens up new opportunities for solving urgent problems of the gas industry. They include the so-called tasks of the upper level of management, aimed at studying the features of the operation of the Unified Gas Supply System (UGSS) of Russia as a whole under normal and peak operating modes. The solution of these problems involves the search for systemic control actions that minimize some system-wide objective function under restrictions that characterize production capacities and loading of large objects and subsystems of the UGSS. In these tasks, the technological nuances of the operation of individual technological objects fade into the background. They focus on the analysis of the sufficiency of the system's production capacity to meet the demand for gas within Russia and fulfill export contractual obligations. The article considers a model of the UGSS gas transmission system of Russia, designed to create, on its basis, a calculation tool for solving problems of this class. The proposed model does not claim to replace the existing and developing methods of GTS modeling when it comes to the study of individual subsystems of the UGSS, but differs in two features. Firstly, unlike the flow models traditionally used in these tasks, it allows taking into account significant technological features of the UGSS (for example, the effects of changes in the volumes of gas accumulated in the GTS). Secondly, unlike more detailed hydraulic models, the proposed model in the hands of a specialist is the operational tool for rational solutions searching when controlling the UGSS as a whole through the use of neural network approximations of the main dependencies that characterize the operation of the UGSS subsystems and provide high speed calculations. The article proves the validity of the proposed approach to the neural network modeling of the UGSS. The practical significance of the model is demonstrated by examining the operation of GTS large fragment of UGSS. It is noted that the further development of neural network modeling of the UGSS should be coordinated with the development of software systems designed for detailed modeling of GTS modes.
Bibliography:
1. Sukharev M.G., Samoylov R.V. Analiz i upravlenie statsionarnymi i nestatsionarnymi rezhimami transporta gaza: monogr. – M.: Izd. tsentr RGU nefti i gaza (NIU) im. I.M. Gubkina, 2016. – 397 s.2. Kislenko N.A., Belinskiy A.V., Kazak A.S. Metody, algoritmy i instrumenty modelirovaniya i optimizatsii rezhimov raboty Edinoy sistemy gazosnabzheniya Rossii na osnove tekhnologiy iskusstvennogo intellekta. Chast' 1 // Gazovaya prom-st'. – 2021. – № 9(821). – S. 88–96.
3. Kislenko N.A., Belinskiy A.V., Kazak A.S. Metody, algoritmy i instrumenty modelirovaniya i optimizatsii rezhimov raboty Edinoy sistemy gazosnabzheniya Rossii na osnove tekhnologiy iskusstvennogo intellekta. Chast' 2 // Gazovaya prom-st'. – 2021. – № 10(822). – S. 98–104.
4. Sardanashvili S.A. Raschetnye metody i algoritmy (truboprovodnyy transport gaza). – M.: Neft' i gaz, 2005. – 577 s.
5. Sukharev M.G., Tverskoy I.V. O metodologii primeneniya matematicheskikh modeley neyronnykh setey k problemam neftegazovogo kompleksa // Avtomatizatsiya i informatizatsiya TEK. – 2022. – № 2(583). – S. 28–35. – DOI: 10.33285/2782-604X-2022-2(583)-28-35
6. Sukharev M., Popov R., Balchenko A. Multiple-criteria decision analysis for cross-country gas pipelines // E3S Web of Conf. – 2019. – Vol. 102. Mathematical Models and Methods of the Analysis and Optimal Synthesis of the Developing Pipeline and Hydraulic Systems. – DOI: 10.1051/e3sconf/201910203010
7. Sukharev M.G., Popov R.V., Bal'chenko A.S. Problemy mnogokriterial'nogo upravleniya mnogonitochnymi koridorami gazoprovodov // Matematicheskie modeli i metody analiza i optimal'nogo sinteza razvivayushchikhsya truboprovodnykh i gidravlicheskikh sistem: tr. XV Vseros. nauch. seminara, Irkutsk, 5–11 sent. – Irkutsk: ISEM SO RAN, 2016. – S. 283–304.
8. Sukharev M.G., Tverskoy I.V., Belinskiy A.V. Kriterii effektivnosti i optimal'nosti tekhnologicheskikh rezhimov gazotransportnykh sistem // Matematicheskie modeli i metody analiza i optimal'nogo sinteza razvivayushchikhsya truboprovodnykh i gidravlicheskikh sistem: tr. XII Vseros. nauch. seminara s mezhdunar. uchastiem, Yalta, 20–26 sent. – Irkutsk: ISEM SO RAN, 2010. – S. 334–346.
9. Energoeffektivnye rezhimy gazotransportnykh sistem i printsipy ikh obespecheniya / A.M. Karasevich, M.G. Sukharev, A.V. Belinskiy [i dr.] // Gazovaya prom-st'. – 2012. – № 1(672). – S. 30–34.
10. R Gazprom 2-3.5-647-2012. Metodika ekonomichnogo upravleniya nizkonapornymi rezhimami gazotransportnoy sistemy. – M.: OAO "Gazprom", 2014. – IV, 51 s.
11. R Gazprom 8-017-2020. Optimizatsiya potokov gaza po GTS ESG s uchetom energozatrat na transportirovku pri dispetcherskom upravlenii. – SPb.: Gazprom ekspo, 2020. – V, 31 s.
12. Gusev A.V., Kireev A.Yu. Strukturnyy analiz sostoyaniya i perspektivy razvitiya dispetcherskogo upravleniya ESG RF // Gazovaya prom-st'. – 2019. – № 2(780). – S. 16–22.
13. Otsenka potentsiala energosberezheniya v magistral'nom transporte gaza: problemy, realizatsiya, perspektivy / G.A. Khvorov, M.N. Matsuk, A.V. Belinskiy [i dr.] // Gazovaya prom-st'. – 2017. – № 7(755). – S. 76–85.
14. Goodfellow I., Bengio Y., Courville A. Deep Learning. – MIT Press, 2016. – XVI, 782 p.
15. Belinskiy A.V. Deep Reinforcement Learning v upravlenii krupnymi inzhenernymi sistemami // Al'manakh "Iskusstvennyy intellekt". – M., 2020.