Scientific and technical journal
«Automation and Informatization of the fuel and energy complex»
ISSN 0132-2222
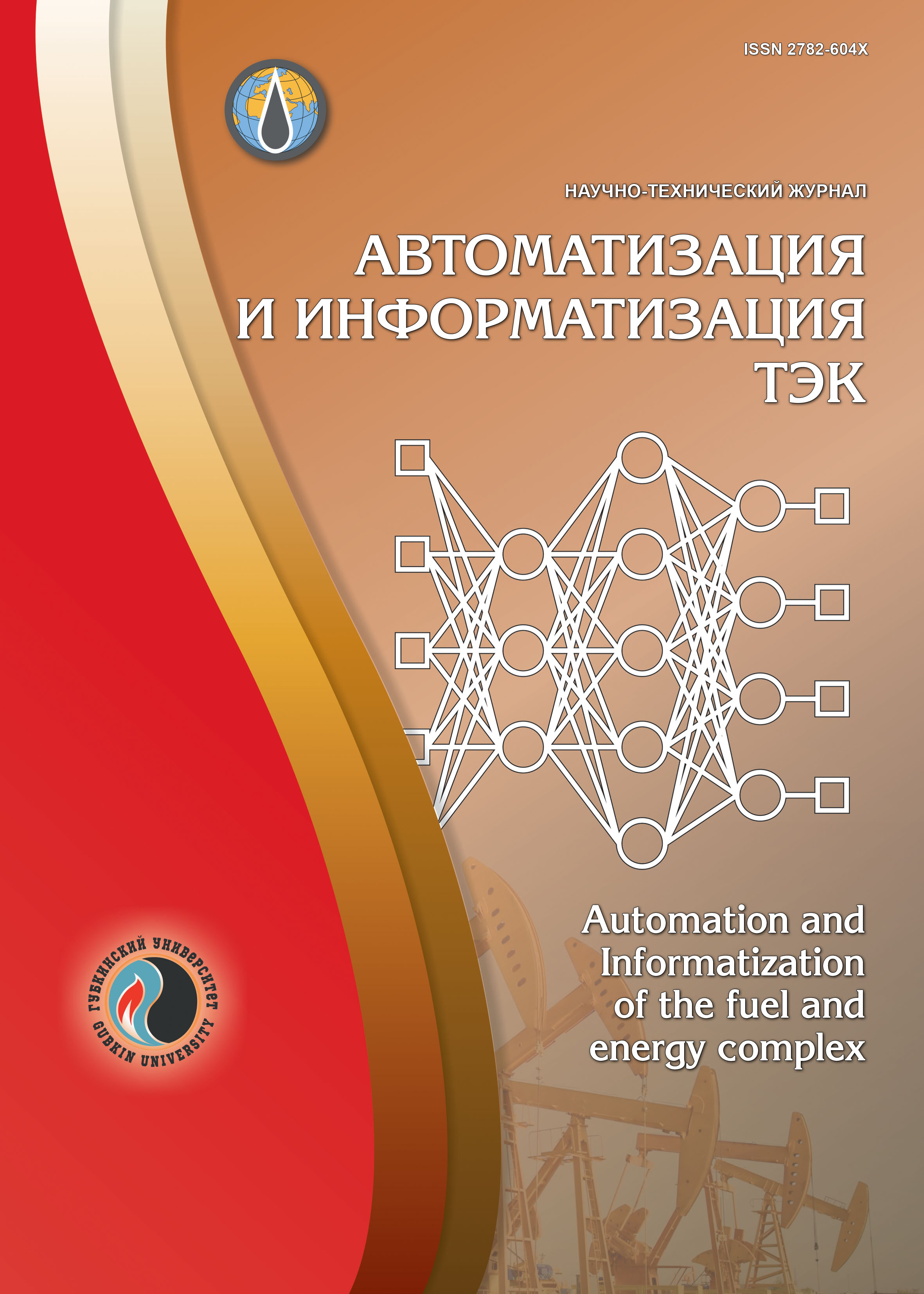
A methodology for constructing inter-well numerical models with tracking of fluid propagation front to assess the dependencies between the operation of production and injection wells
UDC: 620.113+681.121.8
DOI: 10.33285/2782-604X-2023-2(595)-37-50
Authors:











1 NNTC, Novosibirsk, Russia
2 Skolkovo Institute of Science and Technology
3 Novosibirsk State University, Novosibirsk, Russia
4 Gazpromneft STC, St. Petersburg, Russia
5 Skartel (YOTA), Moscow, Russia
Keywords: water-flooding, inverse problem, hydrodynamic model, big data processing
Annotation:
The article presents a methodology for constructing inter-well numerical models with front tracking in order to determine the relationship between the work of producing and injection wells as well as predicting the hydrocarbons potential production rate. To test the methodology, it was tested using a synthetic hydrodynamic model with real field characteristics. An approach of taking into account the impact of various geological-technical measures on the water-flooding process is proposed; a comparative analysis of the results is performed.
Bibliography:
1. Primenenie proksi-modeley gazovykh zalezhey dlya optimizatsii dobychi / Ya.S. Chudin, G.N. Chumakov, I.A. Fedorov [i dr.] // Gazovaya prom-st'. – 2020. – № 4(799). – S. 30–36.2. Sayarpour M. Development and Application of Capacitance/Resistive Models to Water/CO2 Floods: PhD dissertation. – The University of Texas at Austin, 2008. – 236 p. – URL: https://repositories.lib.utexas.edu/bitstream/handle/2152/15357/sayarpourd53148.pdf?sequence=2&isAllowed=y
3. A Capacitance Model to Infer Interwell Connectivity from Production and Injection Rate Fluctuations / A.A. Yousef, P.H. Gentil, J.L. Jensen, L. Lake // SPE Reservoir Evaluation & Engineering. – 2006. – Vol. 9, Issue 6. – P. 630–646. – DOI: 10.2118/95322-PA
4. EUR Assessment Unconventional Assets Using Machine Learning and Distributed Computing Techniques / Zhenyu Guo, Chaohui Chen, Guohua Gao [et al.] // Proc. of the Unconventional Resources Technology Conference, July 24–26, 2017, Austin, Texas, USA. – DOI: 10.15530/urtec-2017-2659996
5. Zhenyu Guo, Reynolds A.C., Hui Zhao. A physics-based data-driven model for history-matching, prediction and characterization of waterflooding performance // Proc. of the SPE Reservoir Simulation Conference, Feb. 20–22, 2017, Montgomery, Texas, USA. – 2017. – DOI: 10.2118/182660-MS
6. History matching and production optimization of water flooding based on a data-driven interwell numerical simulation model // Hui Zhao, Ying Li, Shuyue Cui [et al.] // J. of Natural Gas Science and Engineering. – 2016. – Vol. 31. – P. 48–66. – DOI: 10.1016/j.jngse.2016.02.043
7. Zhenyu Guo, Reynolds A.C., Hui Zhao. Waterflooding optimization with the INSIM-FT data-driven model // Computational Geosciences. – 2018. – Vol. 22. – P. 745–761. – DOI: 10.1007/s10596-018-9723-y
8. INSIM: A Data-Driven Model for History Matching and Prediction for Waterflooding Monitoring and Management with a Field Application / Hui Zhao, Zhijiang Kang, Xiansong Zhang [et al.] // SPE Reservoir Simulation Symp., Houston, Feb. 23–25. – 2015. – DOI: 10.2118/173213-MS
9. End-to-end neural network approach to 3D reservoir simulation and adaptation / E. Illarionov, P. Temirchev, D. Voloskov [et al.] // J. of Petroleum Science and Engineering. – 2022. – Vol. 208, Part A. – DOI: 10.1016/j.petrol.2021.109332
10. Reduced order reservoir simulation with neural-network based hybrid model / P. Temirchev, A. Gubanova, R. Kostoev [et al.] // SPE Russian Petroleum Technology Conf., Oct. 22–24, 2019, Moscow, Russia. – 2019. – DOI: 10.2118/196864-MS
11. Deep neural networks predicting oil movement in a development unit / P. Temirchev, M. Simonov, R. Kostoev [et al.] // J. of Petroleum Science and Engineering. – 2020. – Vol. 184. – DOI: 10.1016/j.petrol.2019.106513
12. Soprovozhdenie razrabotki neftyanykh mestorozhdeniy s ispol'zovaniem modeley CRM: monogr. / S.V. Stepanov, A.D. Bekman, A.A. Ruchkin, T.A. Pospelova. – Tyumen': IPTs "Ekspress", 2021. – 300 s.
13. Buckley S.E., Leverett M.C. Mechanism of fluid displacements in sands // Transactions of the AIME. – 1942. – Vol. 146, Issue 01. – P. 107–116. – DOI: 10.2118/942107-G
14. Godunov S.K. Raznostnyy metod chislennogo rascheta razryvnykh resheniy uravneniy gidrodinamiki // Mat. sb. – 1959. – T. 47(89), № 3. – S. 271–306.
15. Emerick A.A., Reynolds A.C. History-Matching Production and Seismic Data in a Real Field Case Using the Ensemble Smoother with Multiple Data Assimilation // SPE Reservoir Simulation Symp., The Woodlands, Texas, Feb. 18–20. – 2013. – DOI: 10.2118/163675-MS
16. Bailer-Jones C.A.L., Mackay D., Withers P.J. A Recurrent Neural Network for Modelling Dynamical System // Network Computation in Neural System. – 1998. – Vol. 9, Issue 4. – P. 531–547. – DOI: 10.1088/0954-898X_9_4_008
17. Kontrol' razvitiya tekhnogennykh treshchin avtoGRP pri podderzhanii plastovogo davleniya na mestorozhdeniyakh OOO "RN-Yuganskneftegaz" / V.A. Baykov, I.M. Burakov, I.D. Latypov [i dr.] // Neft. khoz-vo. – 2012. – № 11. – S. 30–33.