Scientific and technical journal
«Automation and Informatization of the fuel and energy complex»
ISSN 0132-2222
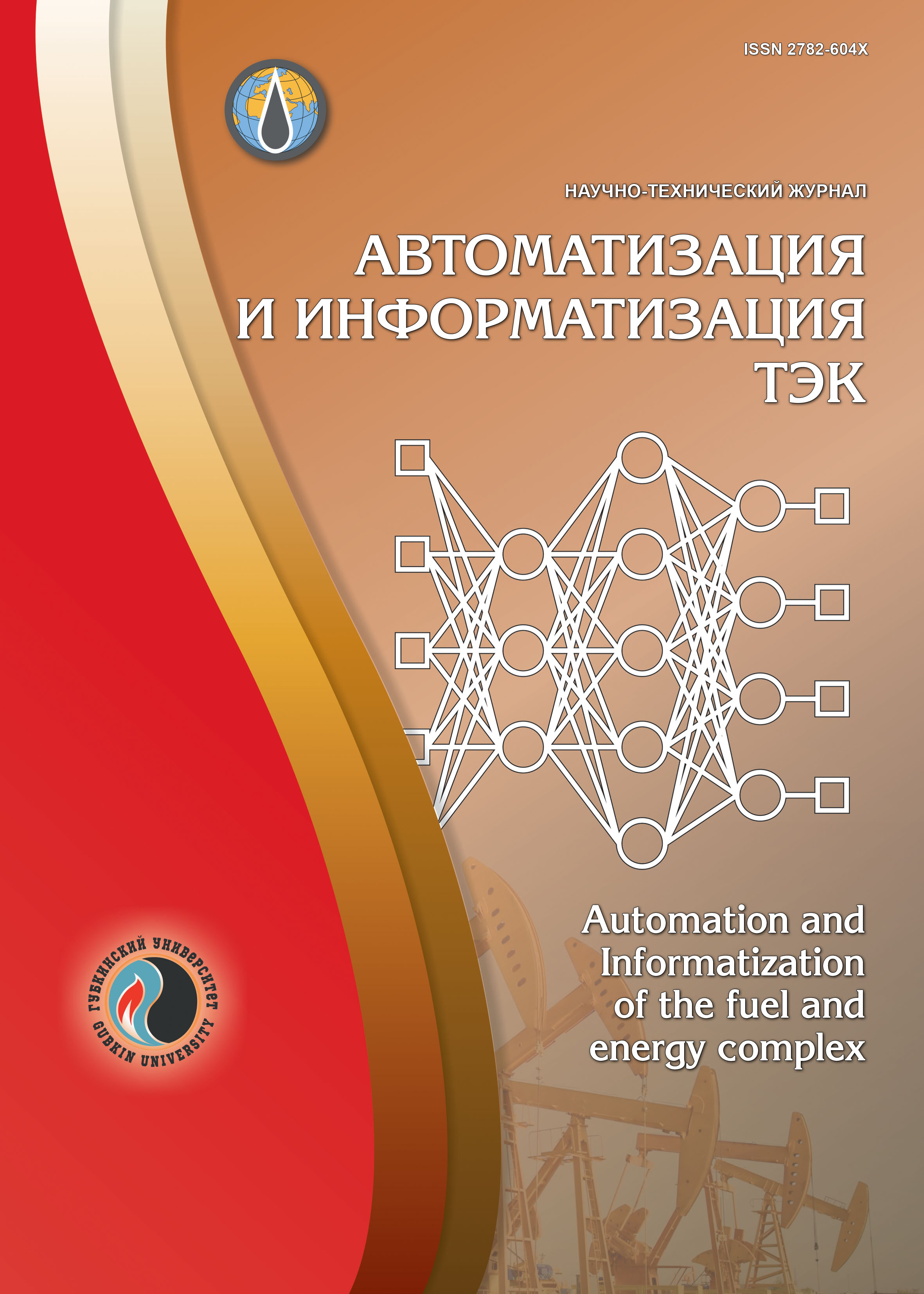
Complex modeling of the oil production processes: analytical review
UDC: 681.5:622.276
DOI: 10.33285/2782-604X-2023-2(595)-51-62
Authors:





1 Peter the Great St. Petersburg Polytechnic University, St. Petersburg, Russia
Keywords: simulation modeling, modeling of oil production processes, predictive models of oil production, multilevel oil production model
Annotation:
The world scientific community is aware of a lot of oil production mathematical models that solve the problems of predicting the oil flow rate, as well as for debugging the work of structures, technological accounting, and balance sheet profit prediction. However, the presence of many practices applied is a problem for researchers due to the lack of a generally accepted classification of the models used. Thus, this work aims to review the existing practices of modeling oil production processes, considering the applicability of certain types of tools for different levels of modeling. Within the study, the model classification was carried out according to the following criteria: the modeling level, the model type, modeling tools, multilevel solutions (the possibility of making decisions at different modeling levels), the availability of the real field-testing, modeling tasks. The most promising approach in terms of the prediction accuracy and duration is simulation modeling that takes into account the formation structure and geological indicators. Statistical models and machine learning models show an average prediction accuracy, which is caused by limited capabilities to account for the characteristics of the oil production process. This study is an analytical basis for the further research and development in the field of modeling oil production processes.
Bibliography:
1. Hybrid Simulation as a Key Tool for Socio-economic Systems Modeling / A.M. Gintciak, M.V. Bolsunovskaya, Zh.V. Burlutskaya, A.A. Petryaeva // Lecture Notes in Networks and Systems. Vol. 442. Int. Conf. System Analysis in Engineering and Control, St. Petersburg, Russia, Oct. 13-14, 2021. – Cham: Springer, 2022. – P. 262–272. – DOI: 10.1007/978-3-030-98832-6_232. Matematicheskoe modelirovanie ekspluatatsionnoy skvazhiny v protsesse optimizatsii neftedobychi / S.G. Voronin, D.A. Kurnosov, M.I. Korabel'nikov [i dr.] // Vestn. Yuzhno-Ural'skogo gos. un-ta. Ser.: Energetika. – 2005. – № 9(49). – S. 70–74.
3. Analytical model for pressure and rate analysis of multi-fractured horizontal wells in tight gas reservoirs / Shan Huang, Yuedong Yao, Ruoyu Ma, Jingwei Wang // J. of Petroleum Exploration and Production Technology. – 2019. – Vol. 9, Issue 1. – P. 383–396. – DOI: 10.1007/s13202-018-0462-3
4. Analytical model for production performance analysis of multi-fractured horizontal well in tight oil reservoirs / Jinghao Ji, Yuedong Yao, Shan Huang [et al.] // J. of Petroleum Science and Engineering. – 2017. – Vol. 158. – P. 380–397. – DOI: 10.1016/j.petrol.2017.08.037
5. Oil production forecast models based on sliding window regression / A. Davtyan, A. Rodin, I. Muchnik, A. Romashkin // J. of Petroleum Science and Engineering. – 2020. – Vol. 195. – DOI: 10.1016/j.petrol.2020.107916
6. Male F. Using a segregated flow model to forecast production of oil, gas, and water in shale oil plays // J. of Petroleum Science and Engineering. – 2019. – Vol. 180. – P. 48–61. – DOI: 10.1016/j.petrol.2019.05.010
7. Production decline analysis in the Eagle Ford / F. Male, M. Marder, J. Browning [et al.] // SPE/AAPG/SEG Unconventional Resources Technology Conf., San Antonio, Texas, USA, Aug. 1–3, 2016. – OnePetro, 2016. – DOI: 10.15530/urtec-2016-2458308
8. Forecasting production from Bakken and three forks wells using a segregated flow model / F. Male, A. Gherabati, J. Browning [et al.] // SPE/AAPG/SEG Unconventional Resources Technology Conf., Austin, Texas, USA, July 24–26, 2017. – OnePetro, 2017. – DOI: 10.15530/URTEC-2017-2666809
9. Classical and machine learning modeling of crude oil production in Nigeria: Identification of an eminent model for application / Ch.P. Obite, A. Chukwu, D. Chekwube [et al.] // Energy Reports. – 2021. – Vol. 7. – P. 3497–3505. – DOI: 10.1016/j.egyr.2021.06.005
10. Siddhamshetty P., Kwon J.S.-II. Model-based feedback control of oil production in oil-rim reservoirs under gas coning conditions // Computers & Chemical Engineering. – 2018. – Vol. 112. – P. 112–120. – DOI: 10.1016/j.compchemeng.2018.02.001
11. Nashawi I.S., Malallah A., Al-Bisharah M. Forecasting World Crude Oil Production Using Multicyclic Hubbert model // Energy & Fuels. – 2010. – Vol. 24, Issue. 3. – P. 1788–1800. – DOI: 10.1021/ef901240p
12. Dynamic response mechanism of borehole breathing in fractured formations / Hongwei Yang, Reyu Gao, Jun Li [et al.] // Energy Reports. – 2022. – Vol. 8. – P. 3360–3374. – DOI: 10.1016/j.egyr.2022.02.148
13. A new SAGD comprehensive multi-stage model for oil production using a concave parabola geometry / Yunfei Guo, Huiqing Liu, Yabin Feng [et al.] // J. of Petroleum Science and Engineering. – 2022. – Vol. 208, Part A. – DOI: 10.1016/j.petrol.2021.109321
14. Wei Liu, Wei David Liu, Jianwei Gu. Forecasting oil production using ensemble empirical model decomposition based Long Short-Term Memory neural network // J. of Petroleum Science and Engineering. – 2020. – Vol. 189. – DOI: 10.1016/j.petrol.2020.107013
15. Okhlopkov G.N. Razrabotka modeli prognozirovaniya ob"emov dobychi i potrebleniya nefti i prirodnogo gaza // Regional'naya ekonomika: teoriya i praktika. – 2009. – № 14. – S. 30–32.
16. Probabilistic assessment of shale gas production and water demand at Xiuwu Basin in China / Youqin Zou, Changbing Yang, Daishe Wu [et al.] // Applied Energy. – 2016. – Vol. 180. – P. 185–195. – DOI: 10.1016/j.apenergy.2016.07.099
17. Brohi I., Pooladi-Darvish M., Aguilera R. Modeling fractured horizontal wells as dual porosity composite reservoirs – application to tight gas, shale gas and tight oil cases // SPE Western North American Region Meeting, Anchorage, Alaska, USA, May 7–11, 2011. – OnePetro, 2011. – DOI: 10.2118/144057-MS
18. Sadov V.B. Otsenka parametrov neftedobychi i upravlenie nasosnoy ustanovkoy s ispol'zovaniem dinamogramm // Vestn. Yuzhno-Ural'skogo gos. un-ta. Ser.: Komp'yuternye tekhnologii, upravlenie, radioelektronika. – 2013. – T. 13, № 2. – S. 33–41.
19. A hybrid semi-analytical model for production from heterogeneous tight oil reservoirs with fractured horizontal well / Linsong Cheng, Sidong Fang, Yonghui Wu [et al.] // J. of Petroleum Science and Engineering. – 2017. – Vol. 157. – P. 588–603. – DOI: 10.1016/j.petrol.2017.07.008
20. Al Rbeawi S., Tiab D. Transient pressure analysis of a horizontal well with multiple inclined hydraulic fractures using type-curve matching // SPE Int. Symposium and Exhibition on Formation Damage Control, Lafayette, Louisiana, USA, Feb. 15–17, 2012. – OnePetro, 2012. – DOI: 10.2118/149902-MS
21. Virstyuk A.Yu., Mishina V.S. Primenenie regressionnogo analiza dlya otsenki effektivnosti raboty neftyanykh skvazhin s parafinistoy neft'yu // Izv. Tomskogo politekhn. un-ta. Inzhiniring georesursov. – 2020. – T. 331, № 1. – S. 117–124. – DOI: 10.18799/24131830/2020/1/2453
22. The design of high-viscosity oil reservoir model based on the inverse problem solution / M.G. Persova, Yu.G. Soloveichik, D.V. Vagin [et al.] // J. of Petroleum Science and Engineering. – 2021. – Vol. 199. – DOI: 10.1016/j.petrol.2020.108245
23. Jin Lang, Jiao Zhao. Modeling and optimization for oil well production scheduling // Chinese J. of Chemical Engineering. – 2016. – Vol. 24, Issue 10. – P. 1423–1430. – DOI: 10.1016/j.cjche.2016.04.050
24. Bogachkova L.Yu. O primenenii modeli Pindayka k analizu sostoyaniya i perspektiv razvitiya nedropol'zovaniya v Rossii (na primere neftedobychi) // Ekonomicheskaya nauka sovremennoy Rossii. – 2002. – № 1. – S. 38–50.
25. Efendieva A.T.K. Matematicheskaya model' khaoticheskogo povedeniya v protsessakh neftedobychi // Byul. nauki i praktiki. – 2016. – № 7(8). – S. 54–58. – DOI: 10.5281/zenodo.58089
26. Apergis N., Ewing B.T., Payne J.E. A time series analysis of oil production, rig count and crude oil price: Evidence from six U.S. oil producing regions // Energy. – 2016. – Vol. 97. – P. 339–349. – DOI: 10.1016/j.energy.2015.12.028
27. Brandt A.R., Plevin R.J., Farrell A.E. Dynamics of the oil transition: modeling capacity, depletion, and emissions // Energy. – 2010. – Vol. 35, Issue 7. – P. 2852–2860. – DOI: 10.1016/j.energy.2010.03.014
28. Scientific engineering as the basis of modeling processes in field development / M.M. Khasanov, A.N. Sitnikov, A.A. Pustovskikh [et al.] // Georesources. – 2018. – Vol. 20, No. 3. – P. 142–148. – DOI: 10.18599/grs.2018.3.142-148
29. Podkhody k modelirovaniyu gidrorazryva plasta i napravleniya ikh razvitiya / M.M. Khasanov, G.V. Paderin, E.V. Shel' [i dr.] // Neft. khoz-vo. – 2017. – № 12. – S. 37–41. – DOI: 10.24887/0028-2448-2017-12-37-41