Научно-технический журнал
«Onshore and offshore oil and gas well construction»
ISSN 0130-3872
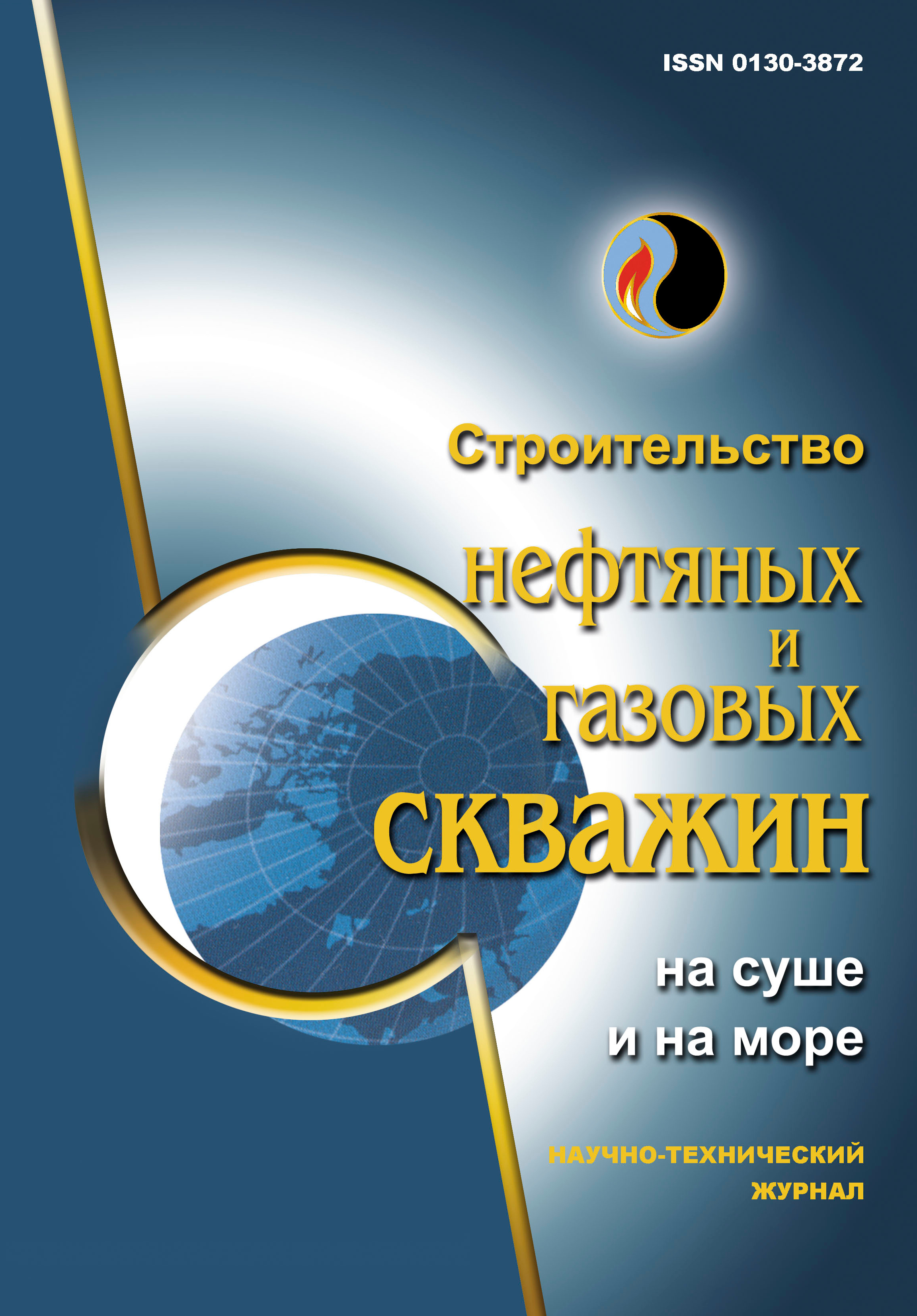
Increasing the efficiency of operation of complicated oil wells using intelligent algorithms
UDC: 622.276.5
DOI: 10.33285/0130-3872-2023-8(368)-50-58
Authors:






1 ANK Bashneft, Ufa, Russia
2 Bashneft-Dobycha, Ufa, Russia
3 RN-BashNIPIneft, Ufa, Russia
4 Ufa State Oil Technical University, Ufa, Russia
Keywords: well, oil production, complications, supply of chemicals, intelligent algorithms, dynamometer charts, gradient boosting, machine learning, forecast
Annotation:
Intelligent algorithms have been proposed that have shown a high quality of work on large data arrays and allow real-time improvement of oil field operation efficiency. The operation of a complicated fund of producing oil wells in PJSC ANK "Bashneft" is analyzed. Statistical data on failures of wells equipped with sucker rod pumping units (SRPU) and electrical submersible pumps (ESP) are presented. The experience of preventing complications by supplying chemical reagents through small diameter pipelines is summarized. The application of an integrated approach to work with a complicated well stock using digital tools is shown. Algorithms for analyzing dynamometer charts and wattmeter charts are considered, which make it possible to calculate the actual parameters of the sucker rod pumping units (SRPU) operation, timely determine deviations in the pump operation and identify broken belts. The implementation of a set of measures over the past three years has made it possible to increase the reliability of wells equipped with electrical submersible pumps (ESP) by 15 %, and with sucker rod pumping units (SRPU) by 22 %.
Bibliography:
1. Enikeev R.M., Zdol'nik S.E., Garifullin A.R. Bashneft'-Dobycha: opyt raboty s oslozhnennym fondom skvazhin // Neftegazovaya vertikal'. – 2015. – № 19. – S. 70–73.2. Vyvod na rezhim skvazhin, ekspluatiruemykh ustanovkami elektrotsentrobezhnykh i skvazhinnykh shtangovykh nasosov, s primeneniem metodov mashinnogo obucheniya i tsifrovykh dvoynikov / A.A. Pashali, D.V. Sil'nov, A.S. Topol'nikov [i dr.] // Neft. khoz-vo. – 2021. – № 7. – S. 112–117. – DOI: 10.24887/0028-2448-2021-7-112-117
3. Tsifrovoy dvoynik skvazhiny kak instrument tsifrovizatsii vyvoda skvazhin na rezhim v PAO ANK "Bashneft'" / A.A. Pashali, A.V. Kolonskikh, R.S. Khalfin [i dr.] // Neft. khoz-vo. – 2021. – № 3. – S. 80–85. – DOI: 10.24887/0028-2448-2021-3-80-84
4. Avtomatizirovannaya sistema interpretatsii otkloneniy po dinamogrammam na osnove sredstv mashinnogo obucheniya pri ekspluatatsii skvazhinnykh shtangovykh nasosov / M.G. Volkov, D.V. Sil'nov, A.S. Topol'nikov [i dr.] // Neft. khoz-vo. – 2021. – № 4. – S. 102–105. – DOI: 10.24887/0028-2448-2021-4-102-105
5. Razrabotka tekhnologii instrumental'nogo kontrolya kachestva neftepromyslovykh khimicheskikh reagentov v PAO ANK "Bashneft'" / A.V. Katermin, A.A. Palaguta, L.R. Mikhaylova [i dr.] // Neft. khoz-vo. – 2021. – № 9. – S. 96–99. – DOI: 10.24887/0028-2448-2021-9-96-99
6. Garifullin I.Sh. Effektivnost' primeneniya spetsial'nogo pogruzhnogo kabel'nogo ustroystva dlya preduprezhdeniya asfal'tosmoloparafinovykh otlozheniy v skvazhinakh // Neft. khoz-vo. – 2005. – № 12. – S. 92–95.
7. Shaydakov V.V., Chernova K.V., Penzin A.V. Sovremennye khimicheskie metody nasosnogo dozirovaniya v neftedobyche. – M.-Vologda: Infra-Inzheneriya, 2018. – 120 s.
8. Experimental Injection set-ups for downhole chemical dosing / B.C. Buñing, M.T. Noriega, Z.F. Sarmiento, R.C.M. Malate // Proc. of World Geothermal Congress 2000, Kyushu – Tohoku, Japan, May 28 – June 10, 2000. – P. 3033–3038.
9. Capillary Tube Technology in Downhole Pressure Acquisition and its Application in Campos Basin / P.G. Cassarà, E. Almanza, J.C. Burgoa, P. Ringgenberg // IADC/SPE Drilling Conf., March 4–6, 2008, Orlando, Florida, USA. – DOI: 10.2118/111465-MS
10. Salakhutdinova K.I., Lebedev I.S., Krivtsova I.E. Algoritm gradientnogo bustinga derev'ev resheniy v zadache identifikatsii programmnogo obespecheniya // Nauch.-tekhn. vestn. inform. tekhnologiy, mekhaniki i optiki. – 2018. – T. 18, № 6. – S. 1016–1022. – DOI: 10.17586/2226-1494-2018-18-6-1016-1022
11. Sevast'yanov A.Yu., Kucheryavykh I.V. Povyshenie tochnosti rascheta debita po plunzhernoy dinamogramme za schet ucheta raboty svobodnogo gaza i intellektual'nogo rascheta utechki zhidkosti // Inzhenernaya praktika. – 2013. – № 6. – S. 150–153.
12. Correlation analysis of spatial time series datasets: A filter-and-refine approach / Pusheng Zhang, Yan Huang, S. Shekhar, V. Kumar // Advances in Knowledge Discovery and Data Mining: Proc. of 7th Pacific-Asia Conference, PAKDD 2003, Seoul, Korea, April 30 – May 2, 2003. – Springer Berlin Heidelberg, 2003. – P. 532–544. – DOI: 10.1007/3-540-36175-8_53
13. Comparison of correlation analysis techniques for irregularly sampled time series / K. Rehfeld, N. Marwan, J. Heitzig, J. Kurths // Nonlinear Processes in Geophysics. – 2011. – Vol. 18, Issue 3. – P. 389–404. – DOI: 10.5194/npg-18-389-2011
14. Crone S.F., Kourentzes N. Feature selection for time series prediction – A combined filter and wrapper approach for neural networks // Neurocomputing. – 2010. – Vol. 73, Issue 10-12. – P. 1923–1936. – DOI: 10.1016/j.neucom.2010.01.017
15. Artificial neural networks for non-stationary time series / Tae Yoon Kim, Kyong Joo Oh, Chiho Kim, Jong Doo Do // Neurocomputing. – 2004. – Vol. 61. – P. 439–447. – DOI: 10.1016/j.neucom.2004.04.002
16. Zhang G.P. An investigation of neural networks for linear time-series forecasting // Computers & Operations Research. – 2001. – Vol. 28, Issue 12. – P. 1183–1202. – DOI: 10.1016/S0305-0548(00)00033-2
17. Mehrmolaei S., Keyvanpour M.R. Time series forecasting using improved ARIMA // 2016 Artificial Intelligence and Robotics, Qazvin, Iran, Apr. 09, 2016. – IEEE, 2016. – P. 92–97. – DOI: 10.1109/RIOS.2016.7529496
18. Goryachkin B.S., Kitov M.A. Komp'yuternoe zrenie // E-scio. – 2020. – № 9(48). – S. 317–345.
19. Osobennosti raboty svertochnykh neyronnykh setey / V.O. Skripachev, M.V. Guyda, N.V. Guyda, A.O. Zhukov // Int. J. of Open Information Technologies. – 2022. – Vol. 10, No. 12. – P. 53–61.