Научно-технический журнал
«Onshore and offshore oil and gas well construction»
ISSN 0130-3872
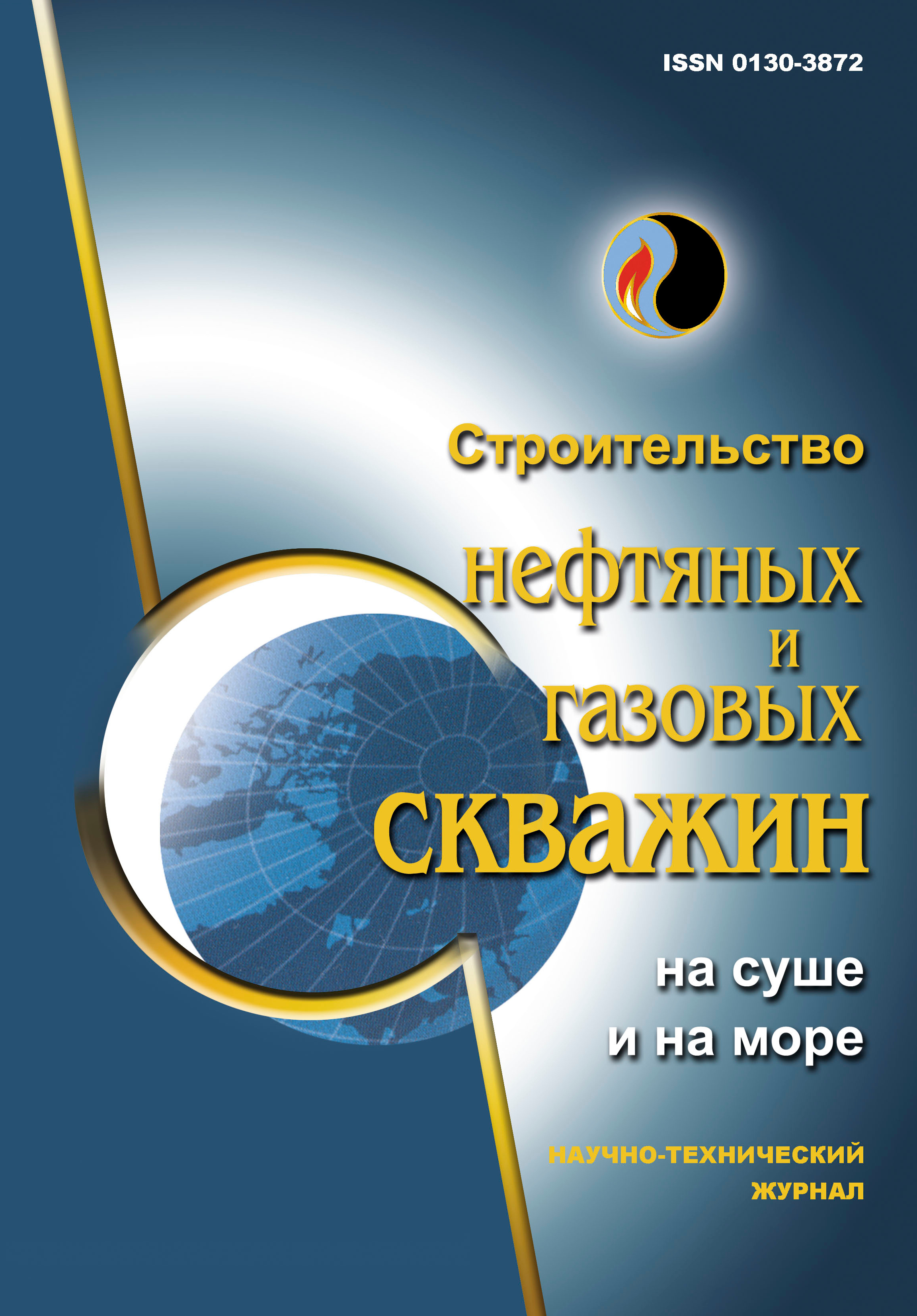
Analysis of the experience of machine learning methods application when drilling oil and gas wells
UDC: 622.24
DOI: 10.33285/0130-3872-2023-9(369)-15-24
Authors:



1 St. Petersburg Mining University, St. Petersburg, Russia
Keywords: well drilling, machine learning, neural networks, gas-water-oil manifestations, drill string sticking, loss of drilling fluid circulation, well logging, ROP
Annotation:
The article analyzes the world experience in using various machine learning methods in the oil and gas industry to optimize the process of drilling oil and gas wells. The well-known methods of machine learning are considered. The spheres of their application are described. According to the results of the analysis, a method of its solution is correlated with each problem under consideration. Today, companies are beginning to actively involve new deposits, often with complex and unpredictable geology in economic turnover. It causes an increase of the cost of all reserves development stages, from exploration to exploitation. At the same time, the most significant capital expenditures fall on the well construction process, which consumes about 40% of the total project cost. The estimated drilling costs can increase significantly due to complications and accidents, the probability of which is proportional to the depth of the well and the degree of the geological section uncertainty. The use of artificial intelligence and machine learning, if implemented correctly, could increase the volume, quality and, as a result, the profitability of production.
Bibliography:
1. Deep learning and time-series analysis for the early detection of lost circulation incidents during drilling operations / M. Aljubran, J. Ramasamy, M. Albassam, A. Magana-Mora // IEEE Access. – 2021. – Vol. 9. – P. 76833–76846. – DOI: 10.1109/ACCESS.2021.30825572. Drilling problems forecast system based on neural network / S. Borozdin, A. Dmitrievsky, N. Eremin [et al.] // SPE Annual Caspian Technical Conf., Oct. 21–22, 2020, Virtual. – DOI:10.2118/202546-MS
3. URL: https://catboost.ai
4. Application of machine learning to accidents detection at directional drilling / E. Gurina, N. Klyuchnikov, A. Zaytsev [et al.] // J. of Petroleum Science and Engineering. – 2020. – Vol. 184. – P. 106519. – DOI: 10.1016/j.petrol.2019.106519
5. Forecasting the abnormal events at well drilling with machine learning / E. Gurina, N. Klyuchnikov, K. Antipova, D. Koroteev // Applied Intelligence. – 2022. – Vol. 52. – P. 9980–9995. – DOI: 10.1007/s10489-021-03013-x
6. Making the black-box brighter: interpreting machine learning algorithm for forecasting drilling accidents / E. Gurina, N. Klyuchnikov, K. Antipova, D. Koroteev // J. of Petroleum Science and Engineering. – 2022. – Vol. 218. – P. 111041. – DOI: 10.1016/j.petrol.2022.111041
7. Real-Time Well Log Prediction from Drilling Data Using Deep Learning / R. Kanfar, O. Shaikh, M. Yousefzadeh, T. Mukerji // Int. Petroleum Technology Conf., Jan. 13–15, 2020, Dhahran, Kingdom of Saudi Arabia. – DOI: 10.2523/IPTC-19693-MS
8. Konik L. "NOVATEK" snizil avariynost' bureniya pri pomoshchi AI. – 2022. – URL: https://www.comnews.ru/content/219388/2022-03-23/2022-w12/novatek-snizil-avariynost-bureniya-pri-pomoschi-ai
9. URL: https://www.lightgbm.readthedocs.io
10. Optimizing drilling wells and increasing the operation efficiency using digital twin technology / M.G. Mayani, T. Baybolov, R. Rommetveit [et al.] // IADC/SPE Int. Drilling Conf. and Exhibition, March 3–5, 2020, Galveston, Texas, USA. – DOI: 10.2118/199566-MS
11. Six years operating a real time drilling problem detection software in deepwater environments: Results and challenges / F.R. da Silva, M. de Souza Cruz, B. Barduchi [et al.] // SPE Latin American and Caribbean Petroleum Engineering Conf., July 27–31, 2020, Virtual. – DOI: 10.2118/199077-MS
12. Mashinnoe obuchenie v Python. – URL: https://www.scikit-learn.ru
13. Qodirov S., Shestakov A. Development of Artificial Neural Network for Predicting Drill Pipe Sticking in Real-Time Well Drilling Process // 2020 Global Smart Industry Conference (GloSIC), Nov. 17–19, 2020, Chelyabinsk, Russia. – DOI: 10.1109/glosic50886.2020.9267873
14. Ob uvelichenii produktivnogo vremeni bureniya neftegazovykh skvazhin s ispol'zovaniem metodov mashinnogo obucheniya / A.N. Dmitrievskiy, A.G. Sboev, N.A. Eremin [i dr.] // Georesursy. – 2020. – T. 22, № 4. – S. 79–85. – DOI: 10.18599/grs.2020.4.79-85
15. Tsifrovoy neftegazovyy kompleks Rossii / A.N. Dmitrievskiy, N.A. Eremin, D.S. Filippova, E.A. Safarova // Georesursy. – 2020. – T. 22, № S. – S. 32–35. – DOI: 10.18599/grs.2020.SI.32-35
16. Tsifrovye tekhnologii stroitel'stva skvazhin. Sozdanie vysokoproizvoditel'noy avtomatizirovannoy sistemy predotvrashcheniya oslozhneniy i avariynykh situatsiy v protsesse stroitel'stva neftyanykh i gazovykh skvazhin / N.A. Eremin, A.D. Chernikov, O.N. Sardanashvili [i dr.] // Delovoy zhurn. Neftegaz.RU. – 2020. – № 4(100). – S. 38–50.
17. Katanov Yu.E. Neyrosetevaya model' prognozirovaniya skorosti i rezhimov bureniya skvazhin v slozhnopostroennykh kollektorakh. – Izv. vuzov. Neft' i gaz. – 2021. – № 1(145). – S. 55–76. – DOI: 10.31660/0445-0108-2021-1-55-76
18. Primenenie metodov iskusstvennogo intellekta dlya vyyavleniya i prognozirovaniya oslozhneniy pri stroitel'stve neftyanykh i gazovykh skvazhin: problemy i osnovnye napravleniya resheniya / A.D. Chernikov, N.A. Eremin, V.E. Stolyarov [i dr.] // Georesursy. – 2020. – T 22, № 3. – S. 87–96. – DOI: 10.18599/grs.2020.3.87-96
19. ACGAN and BN based method for downhole incident diagnosis during the drilling process with small sample data size / Chuan Wang, Jiajun Ma, Hao Jin [et al.] // Ocean Engineering. – 2022. – Vol. 256. – P. 111516. – DOI: 10.1016/j.oceaneng.2022.111516
20. A split–merge clustering algorithm based on the k-nearest neighbor graph / Yan Wang, Yan Ma, Hui Huang [et al.] // Information Systems. – 2023. – Vol. 111. – P. 102124. – DOI: 10.1016/j.is.2022.102124