Scientific and technical journal
«Equipment and technologies for oil and gas complex»
ISSN 1999-6934
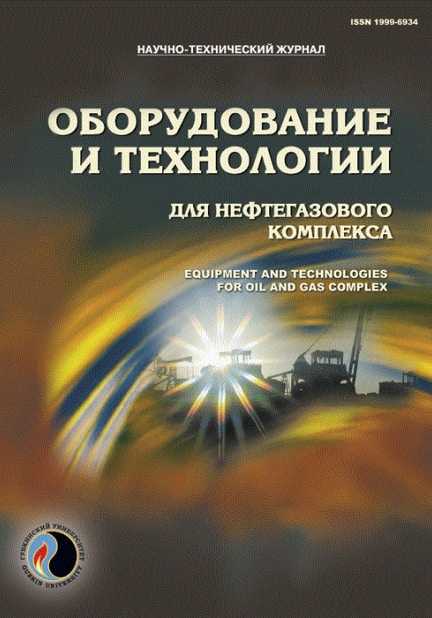
Overview of the application of Bayesian network and artificial intelligence for reducing the number of complications and accidents when developing and operating oil and gas fields
UDC: 622.276.032.85:004.89
DOI: -
Authors:


1 Oil and Gas Research Institute RAS, Moscow, Russia
Keywords: artificial intelligence, Bayesian networks, production organization, database analysis, machine learning, process safety management identification, complications prediction, well drilling, geological and technological information, accident prevention, automated system, well construction, oil production
Annotation:
Each oil and gas company with a safety focus is pursuing process safety management (PSM), which provides better understanding of hazards, comprehensive risk assessment and management, and in-depth studying the experience to improve overall safety and operational performances. Companies often use an incident reporting system, but since it can contain thousands of reports, they rarely take full advantage of them to prevent and reduce future incidents. To address this problem, this study used machine learning and keyword analysis to label and classify 8199 incident reports from an oil and gas company into nine groups identified in the latest version of guidelines published by the Center for Chemical Process Safety. To achieve the optimal solution, two different Bayesian network methods were used, resulting in one and the same map creation. It showed that the total number of incidents is 50 % dependent on the integrity and reliability of files such as drilling logs, meaning that focusing resources on this aspect can reduce the number of incidents by half. In addition, cross-correlation analysis (CCA) was conducted, which confirmed this result and identified measures to improve the company's safety management strategy.
Bibliography:
1. Amin T., Khan F., Imtiaz S. Fault Detection and Pathway Analysis using a Dynamic Bayesian Network // Chemical Engineering Science. – 2019. – Vol. 195. – P. 777–790. – DOI: 10.1016/j.ces.2018.10.0242. Incorporation of inherent safety principles in process safety management / P.R. Amyotte, A.U. Goraya, D.C. Hendershot, F.I. Khan // Process Safety Progress. – 2007. – Vol. 26, Issue 4. – P. 333–346. – DOI: 10.1002/prs.10217
3. Aven T., Krohn B.S. A new perspective on how to understand, assess and manage risk and the unforeseen // Reliability Engineering & System Safety. – 2014. – Vol. 121. – P. 1–10. – DOI: 10.1016/j.ress.2013.07.005
4. Cunlu Zou, Denby K.J., Jianfeng Feng. Granger causality vs. dynamic Bayesian network inference: a comparative study // BMC Bioinformatics. – 2009. – Vol. 10. – DOI: 10.1186/1471-2105-10-122
5. A Dynamic Decision Approach for Risk Analysis in Complex Projects / Xianguo Wu, Yanhong Wang, Limao Zhang [et al.] // J. of Intelligent & Robotic Systems. – 2015. – Vol. 79. – P. 591–601. – DOI: 10.1007/s10846-014-0153-3
6. Usability of accident and incident reports for evidence-based risk modeling – A case study on ship grounding reports / A. Mazaheri, J. Montewka, J. Nisula, P. Kujala // Safety Science. – 2015. – Vol. 76. – P. 202–214. – DOI: 10.1016/j.ssci.2015.02.019
7. Using machine learning and keyword analysis to analyze incidents and reduce risk in oil sands operations / D. Kurian, F. Sattari, L. Lefsrud, Yongsheng Ma // Safety Science. – 2020. – Vol. 130. – P. 104873. – DOI: 10.1016/j.ssci.2020.104873
8. Reporting and Investigating Injuries and Incidents – OHS information for employers, prime contractors and workers. – Government of Alberta, Edmonton, AB, Canada, 2019. – 11 p.
9. Friedman N., Nachman I., Pe'er D. Learning Bayesian Network Structure from Massive Datasets: The "Sparse Candidate" Algorithm // Proceedings of the Fifteenth Conf. on Uncertainty in Artificial Intelligence, Stockholm, Sweden, July 30 – Aug. 1. – 1999. – P. 206–215. – DOI: 10.13140/2.1.1125.2169; Berglund J. After Fukushima: Safety culture and fostering critical thinking // Safety Science. – 2020. – Vol. 124. – P. 104613. – DOI: 10.1016/j.ssci.2020.104613
10. Chemoinformatic investigation of the Chemistry of Cellulose and Lignin Derivatives in Hydrous Pyrolysis / F. Sattari, D. Tefera, K. Sivaramakrishnan [et al.] // Industrial & Engineering Chemistry Research. – 2020. – Vol. 59, Issue 25. – P. 11582–11595. – DOI: 10.1021/acs.iecr.0c01592
11. Uusitalo L. Advantages and challenges of Bayesian networks in environmental modelling // Ecological Modelling. – 2007. – Vol. 203, Issues 3-4. – P. 312–318. – DOI: 10.1016/j.ecolmodel.2006.11.033; Van der Schaaf T., Kanse L. Biases in Incident Reporting Databases: An Empirical Study in the Chemical Process Industry // Safety Science. – 2004. – Vol. 42. – P. 57–67. – DOI: 10.1016/S0925-7535(03)00023-7
12. Berglund J. Why Safety Cultures Degenerate and how to Revive them. – London: Routledge, 2016. – 106 p.
13. Wold T., Laumann K. Safety management systems as communication in an oil and gas producing company // Safety Science. – 2015. – Vol. 72. – P. 23–30. – DOI: 10.1016/j.ssci.2014.08.004
14. Abuashour A.M.B., Hassan Z. Conceptual framework for enhancing safety performance by impact cooperation facilitation, safety communication and work environment: Jordanian hospitals // Sains Humanika. – 2019. – Vol. 11, No. 2-2. – DOI: 10.11113/sh.v11n2-2.1659
15. Eremin N.A., Selenginskiy D.A., Chernikov A.D. Ob ispol'zovanii metodov iskusstvennogo intellekta dlya resheniya zadach v neftegazovoy otrasli // Zolotukhinskie chteniya. Neft', gaz i energetika v Arkticheskom regione: sb. materialov Mezhdunar. nauch.-prakt. konf., Arkhangel'sk, 20–21 apr. 2023 g. – Arkhangel'sk: Severnyy (Arkticheskiy) feder. un-t im. M.V. Lomonosova, 2023. – S. 110–112.
16. Eremin N.A., Selenginskiy D.A. O vozmozhnosti primeneniya metodov iskusstvennogo intellekta v reshenii neftegazovykh zadach // Izv. Tul'skogo gos. un-ta. Nauki o Zemle. – 2023. – № 1. – S. 201–211. – DOI: 10.46689/2218-5194-2023-1-1-201-211