Scientific and technical journal
«Equipment and technologies for oil and gas complex»
ISSN 1999-6934
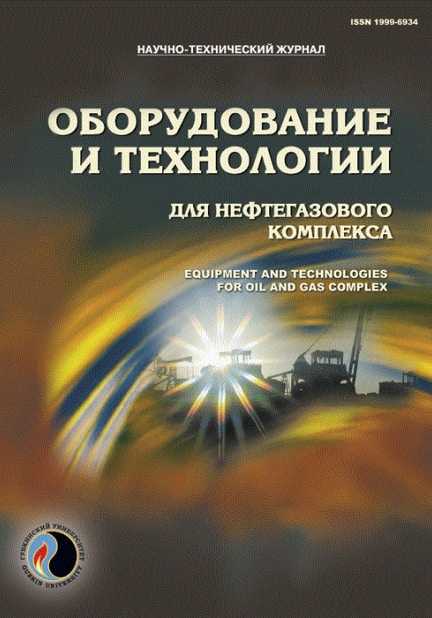
Computer vision and artificial intelligence algorithms for detecting defects in power equipment and pipeline transport facilities
UDC: 004.93:621.3.073.97
DOI: -
Authors:




1 SmartRen, Moscow, Russia
2 National University of Oil and Gas "Gubkin University", Moscow, Russia
3 B&B Industries, Moscow, Russia
Keywords: pipeline transport, power lines, renewable energy sources, solar power plants, wind farms, unmanned aerial vehicles, computer vision, artificial intelligence, convolutional neural networks
Annotation:
This scientific article extensively explores the application of advanced computer vision and artificial intelligence algorithms for detecting defects in various types of energy equipment. The areas of application include power transmission lines, wind energy installations, solar panels, and pipeline transportation objects. The research provides a detailed analysis of the main categories of defects inherent in energy equipment and reviews current methods for detecting these malfunctions. The authors propose an innovative approach by integrating unmanned aerial vehicles and artificial intelligence for effective defect detection. The article presents the technological process of defect detection, describing the details of algorithms specifically designed for a broad spectrum of energy and technological equipment. This approach combines advanced data analysis methods with an automated detection system, ensuring reliability and accuracy in defect identification and contributing to the increased efficiency of maintenance for energy facilities.
Bibliography:
1. The structure healthy condition monitoring and fault diagnosis methods in wind turbines: A review / Wenyi Liu, Baoping Tang, Han Jiguang [et al.] // Renewable and Sustainable Energy Reviews. – 2015. – Vol. 44. – P. 466–472. – DOI: 10.1016/j.rser.2014.12.0052. Yanan Zhang, Avallone F., Watson S. Wind turbine blade trailing edge crack detection based on airfoil aerodynamic noise: An experimental study // Applied Acoustics. – 2022. – Vol. 191. – P. 108668. – DOI: 10.1016/j.apacoust.2022.108668
3. Assessment of methane emissions from the US oil and gas supply chain / R.A. Alvarez, D. Zavala-Araiza1, D.R. Lyon [et al.] // Science. – 2018. – Vol. 361, No. 6398. – P. 186–188. – DOI: 10.1126/science.aar7204
4. Gol'dzon I.A., Zav'yalov A.P., Lopatin A.S. O perspektivakh ispol'zovaniya sistem avtomatizirovannogo kontrolya tekhnicheskogo sostoyaniya oborudovaniya ob"ektov TEK s ispol'zovaniem bespilotnykh tekhnologiy // Avtomatizatsiya, telemekhanizatsiya i svyaz' v neftyanoy promyshlennosti. – 2019. – № 6(551). – S. 25–30. – DOI: 10.33285/0132-2222-2019-6(551)-25-30
5. Budzulyak B.V., Lopatin A.S., Lyapichev D.M. Tekhnicheskoe diagnostirovanie oborudovaniya i truboprovodov ob"ektov neftegazovogo kompleksa s primeneniem innovatsionnykh tekhnologiy // Avtomatizatsiya, telemekhanizatsiya i svyaz' v neftyanoy promyshlennosti. – 2019. – № 11(556). – S. 21–26. – DOI: 10.33285/0132-2222-2019-11(556)-21-26
6. Guseynov K.B., Zaderigolova M.M., Lopatin A.S. Geodinamicheskiy monitoring magistral'nykh gazoprovodov s ispol'zovaniem bespilotnykh letatel'nykh apparatov // Tr. RGU nefti i gaza im. I.M. Gubkina. – 2016. – № 1(282). – S. 80–88.
7. Wind Turbine Blade Defect Detection Based on Acoustic Features and Small Sample Size / Yuefan Zhu, Xiaoying Liu, Shen Li [et al.] // Machines. – 2022. – Vol. 10, Issue 12. – P. 1184. – DOI: 10.3390/machines10121184
8. Eddie Yin Kwee Ng, Jian Tiong Lim. Machine Learning on Fault Diagnosis in Wind Turbines // Fluids. – 2022. – Vol. 7, Issue 12. – P. 371. – DOI: 10.3390/fluids7120371
9. Ying-Yi Hong, Pula R.A. Methods of photovoltaic fault detection and classification: A review // Energy Reports. – 2022. – Vol. 8. – P. 5898–5929. – DOI: 10.1016/j.egyr.2022.04.043
10. Deep learning strategies for automatic fault diagnosis in photovoltaic systems by thermographic image / D. Manno, G. Cipriani, G. Ciulla [et al.] // Energy Conversion and Management. – 2021. – Vol. 241. – P. 114315. – DOI: 10.1016/j.enconman.2021.114315
11. Robust real-time UAV based power line detection and tracking / Guang Zhou, Jinwei Yuan, I-Ling Yen, F. Bastani // IEEE Int. Conf. on Image Processing, Phoenix, AZ, USA, Sept. 25–28. – 2017. – P. 744–748. – DOI: 10.1109/ICIP.2016.7532456
12. Automatic detection of powerlines in UAV remote sensed images / K.N. Ramesh, A.S. Murthy, J. Senthilnath, S.N. Omkar // 2015 Int. Conf. on Condition Assessment Techniques in Electrical Systems (CATCON), Bangalore, India, Dec. 10–12. – 2015. – P. 17–21. – DOI: 10.1109/CATCON.2015.7449501
13. Katrasnik J., Pernus F., Likar B. A survey of mobile robots for distribution power line inspection // IEEE Transactions on power delivery. – 2010. – Vol. 25, Issue 1. – P. 485–493. – DOI: 10.1109/TPWRD.2009.2035427
14. Van Nhan Nguyen, Jenssen R., Roverso D. Automatic autonomous vision-based power line inspection: A review of current status and the potential role of deep learning // Int. J. of Electrical Power & Energy Systems. – 2018. – Vol. 99. – P. 107–120. – DOI: 10.1016/j.ijepes.2017.12.016
15. Review of autonomous inspection technology for power lines using UAVs / Sun Shuangchun, Li Yanlei, Yi Zhenxiao [et al.] // 2021 IEEE Int. Conf. on Electrical Engineering and Mechatronics Technology (ICEEMT), Qingdao, China, July 02–04. – 2021. – P. 481–484. – DOI: 10.1109/ICEEMT52412.2021.9601446
16. A review of UAV power line inspection / Zhaoyang Wang, Qiang Gao, Jianbin Xu, Dahua Li // Advances in Guidance, Navigation and Control: Proceedings of 2020 Int. Conf. on Guidance, Navigation and Control (ICGNC 2020), Tianjin, China, Oct. 23–25, 2020. – Singapore: Springer, 2022. – P. 3147–3159. – DOI: 10.1007/978-981-15-8155-7_263