Scientific and technical journal
«Geology, geophysics and development of oil and gas fields»
ISSN 2413-5011
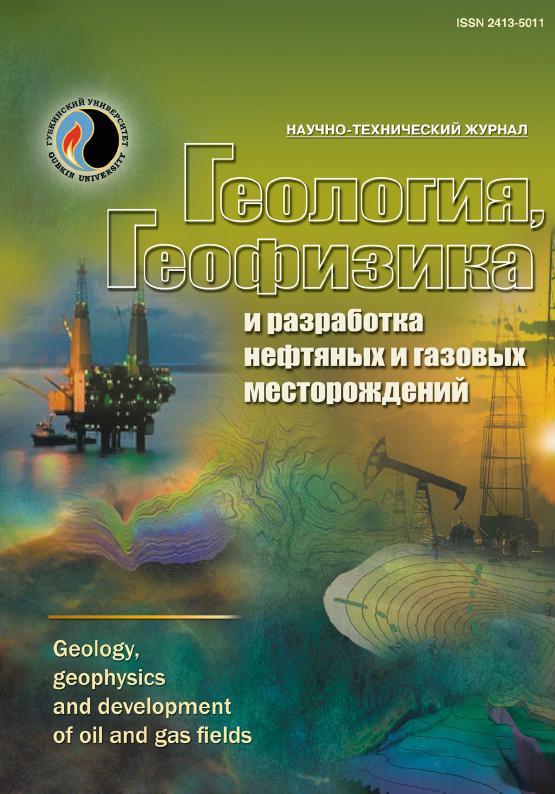
№4 (376), April 2023
Use of generative adversarial networks as a method of facies generation to recreate a reservoir geological heterogeneity
UDC: 622.276
DOI: 10.33285/2413-5011-2023-4(376)-27-35
Authors:
1 Northern (Arctic) Federal University named after M.V. Lomonosov, Arkhangel’sk, Russia
Keywords: data integration, geological uncertainty, reservoir heterogeneity, permeability, neural networks, deep learning, parametrization, Kalman filter, optimization
Annotation:
The application of data integration methods into geological hydrodynamic models to optimize fields’ development has been the subject of intensive research over the past 10 years. Recently, there has been observed a notable progress in the ability of data integration methods to reduce the geological uncertainty of reservoir characteristics and improve field development optimization. However, there are still problems of dynamic data integrate into the model.
The author of the article summarizes the main achievements in the field of water-flooding optimization process using the data integration approach and reviews the previous achievements, including developments in the search for modifications of the Ensemble Kalman Filter (EnKF) and Ensemble Smoother (ES).
The article also presents the results of the development of a deep learning algorithm – a generative adversarial network (GAN) and the demonstration of the process of a synthetic geological model generation:
• without integration of permeability data into the model;
• with the integration of well permeability data into the model.
The results of the work demonstrate the effectiveness of using GAN as facies generation method to recreate a reservoir geological heterogeneity as well as to improve GAN ability to integrate data into a synthetic model.
The author also evaluated the possibility of creating a generative-adversarial network-ensemble smoother pair to improve the closed-loop cycle of an oil field management.
Bibliography:
1. Brouwer D.R., Jansen J.D. Dynamic Optimization of Waterflooding With Smart Wells Using Optimal Control Theory // SPE Journal Society of Petroleum Engineers. – 2004. – Vol. 9. – № 04. – Pp. 391–402.
2. Results of the Brugge Benchmark Study for Flooding Optimization and History Matching / E. Peters [et al.] // SPE Reservoir Simulaton Symposium. – 2010. – DOI: 10.2118/119094-MS
3. Recent Developments Combining Ensemble Smoother and Deep Generative Networks for Facies History Matching / A.A. Emerick [et al.] // Computational Geosciences. – 2020.
4. Generative adversarial networks / I. Goodfellow [et al.] // Commun. – ACM. 2020. – Vol. 63. – № 11. – Pp. 139–144.
5. Closed-Loop Reservoir Management / Jansen J.D. [et al.] // System. – 2009. – DOI: 10.2118/119098-MS
6. Nævdal G., Brouwer D.R., Jansen J.D. Waterflooding using closed-loop control // Comput. Geosci. – 2006. – Vol. 10. – № 1. – Pp. 37–60.
7. Chen Y., Oliver D.S., Zhang D. Efficient Ensemble-Based Closed-Loop Production Optimization // SPE Journal Society of Petroleum Engineers. – 2009. – Vol. 14. – № 04. – Pp. 634–645.
8. Robust Waterflooding Optimization of Multiple Geological Scenarios / van Essen G. [ et al.] // SPE Journal Society of Petroleum Engineers. – 2009. – Vol. 14. – № 01. – Pp. 202–210. – DOI: 10.2118/102913-РА
9. Barros G.D.E., Van den Hof P.M.J., Jansen J.D. Value of information in closed-loop reservoir management // Comput. Geosci. Computational Geosciences. – 2016. – Vol. 20. – № 3. – Pp. 737–749.
10. Kalman R.E. A New Approach to Linear Filtering and Prediction Problems // Journal Basic Eng. ASME. – 1960. – Vol. 82. – № 1. – Pp. 35–45.
11. Khrulenko A., Shchipanov A., Berenblyum R. Conditioning Reservoir Models To Pressure Transients By Iterative Ensemble Smoother. – 2018. – DOI: 10.3997/2214-4609.201802133
12. Gordon N.J., Salmond D.J., Smith A.F.M. Novel approach to nonlinear/non-Gaussian Bayesian state estimation // IEE Proc. F-Radar Signal Process. – 1993. – Vol. 140. – № 2. – Pp. 107–113.
13. Aman B.M. Reservoir History Matching Using Ensemble Kalman Filters with Anamorphosis Transforms: Thesis… degree of Masters of Science. – Saudi Arabia, 2012.
14. Bengio Y., Courville A., Vincent P. Representation Learning: A Review and New Perspectives // IEEE Trans. Pattern Anal. Mach. Intell. – 2013. – Vol. 35. – № 8. – Pp. 1798–1828.
15. Mosser L., Dubrule O., Blunt M.J. DeepFlow: History Matching in the Space of Deep Generative Models. – 2019.
16. Handbook of Mathematical Geosciences / ed. B.S. Daya Sagar, Q. Cheng, F. Agterberg. – Cham: Springer International Publishing, 2018.
17. Sun R., Sessions C. Self-segmentation of sequences: automatic formation of hierarchies of sequential behaviors // IEEE Trans. Syst. Man Cybern. – Part B. – 2000. – Vol. 30. – № 3. – Pp. 403–418.
18. ImageNet Large Scale Visual Recognition Challenge / O. Russakovsky [et al.]. – 2014.
19. Emerick A.A., Reynolds A.C. Ensemble smoother with multiple data assimilation // Comput. Geosci. – 2013. – Vol. 55. – Pp. 3–15.
20. Efficient Ensemble-Based Closed-Loop Production Optimization / Y. Chen [et al.] // SPE Journal. – 2013. – № 14(04). – DOI: 10.2118/112873-MS
21. Guliev R., Zolotukhin A. Field development optimization of waterflooding process using data assimilation methods // IOP Conf. Series. Materiala. Science. Eng. – 2019. – Vol. 700. – № 1. – DOI: 10.1088/1757-899X/700/1/012054