Scientific and economic journal
«Problems of economics and management of oil and gas complex»
ISSN 1999-6942
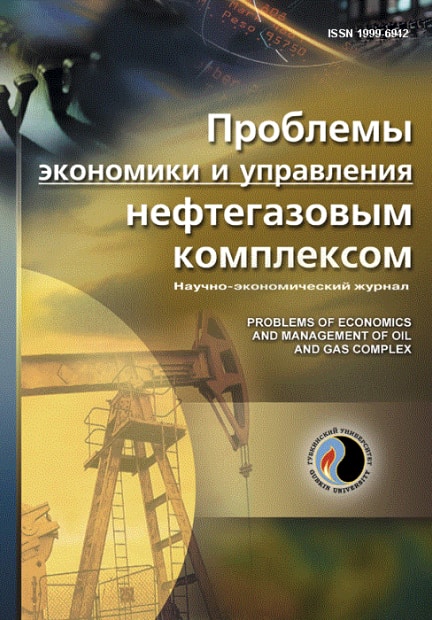
Artificial intelligence in oil and gas production: possibilities and scenario forecast
UDC: 608
DOI: 10.33285/1999-6942-2022-3(207)-38-46
Authors:

1 Grozny State Oil Technical University named after M.D. Millionshchikov, Grozny, Russian Federation
Keywords: artificial intelligence, oil and gas production, upstream, forecast, possibilities, deposits
Annotation:
Artificial intelligence is changing the energy sector of the economy, where the oil and gas production industry is no exception. Oil and gas industry enterprises can be divided into three sectors: upstream, midstream and downstream. The most risky sector of oil and gas production is upstream, which is most in need of using the latest achievements of computer technologies to reduce project risks and uncertainties that directly affect the performance of oil companies. The possibilities of using artificial intelligence for the oil and gas industry in the upstream segment are analyzed. It is proved that the artificial intelligence technology will significantly reduce the project risks of investment decisions by eliminating the subjective component and the duration of the main stages of the new deposits development. The author has developed three scenarios for predicting the development of artificial intelligence technologies in the oil and gas production industry for the next 5, 10 and 20 years. A realistic forecast, which is considered the most likely one, shows that over the next 20 years, artificial intelligence will become a reliable "adviser" when making decisions about the development of new mineral deposits. It is also possible that up to 90 % of decisions will be made based on the recommendations of artificial intelligence, which will significantly increase the marginality of the oil production industry in the long term.
Bibliography:
1. Aksenov K.A., Goncharova N.V. Gibridnoe modelirovanie mul'tiagentnykh protsessov preobrazovaniya resursov: monogr. – M.: Izd. dom Akad. Estestvoznaniya, 2019. – 222 s.2. Dadashev Z.F., Ustinova N.G. Vliyanie iskusstvennogo intellekta na ekonomiku // Epokha nauki. – 2019. – № 18. – S. 53–57. – DOI: 10.24411/2409-3203-2018-11812
3. Informatsionnye tekhnologii v neftegazovoy otrasli. – URL: https://www.tadviser.ru/index.php/Статья:_Информационные_технологии_в_нефтегазовой_отрасли (data obrashcheniya 21.08.2021).
4. Kalenitskiy A.I., Dubrovskiy A.V., Budarova V.A. Tekhnologiya obrabotki rezul'tatov geodezicheskogo obespecheniya 3D seysmorazvedki na territoriyakh mestorozhdeniy nefti i gaza // Vestn. SGUGiT (Sibirskogo gos. un-ta geosistem i tekhnologiy). – 2010. – № 1(12). – S. 21–27.
5. Muzhzhavleva T.V., Kurakova A.V. Problemy organizatsii stran-eksporterov nefti (OPEK) na sovremennom etape // Oeconomia et Jus. – 2018. – № 4. – S. 21–28.
6. Podol'skiy A.K. Primenenie metodov iskusstvennogo intellekta v neftegazovoy promyshlennosti // Sovremennaya nauka. – 2016. – № 3. – S. 33–36.
7. Pronina E.O., Syupova M.S. Kartel'nye soglasheniya: teoriya i praktika // Uchenye zametki TOGU. – 2018. – T. 9, № 1. – S. 196–201.
8. Tcharo Kh., Vorob'ev A.E., Vorob'ev K.A. Tsifrovizatsiya neftyanoy promyshlennosti: bazovye podkhody i obosnovanie "intellektual'nykh" tekhnologiy // Vestn. Evraziyskoy nauki. – 2018. – T. 10, № 2. – S. 77.
9. Shut'ko S.Yu., Kozhevnikova S.D., Shut'ko D.S. Riski i neopredelennosti Upstream // Territoriya Neftegaz. – 2017. – № 1-2. – S. 65–71.
10. Application of machine learning and artificial intelligence in oil and gas industry / A. Sircar, K. Yadav, K. Rayavarapu [et al.] // Petroleum Research. – 2021. – Vol. 6, Issue 4. – P. 379–391. – DOI: 10.1016/j.ptlrs.2021.05.009
11. Barker J.W., Thibeau S. A Critical review of the use of pseudorelative permeabilities for upscaling // SPE Reservoir Engineering. – 1997. – Vol. 12, Issue 2. – P. 138–143. – DOI: 10.2118/35491-PA
12. Brynjolfsson E., Mitchell T. What can machine learning do? Workforce implication // Science. – 2017. – Vol. 358, Issue 6370. – P. 1530–1534. – DOI: 10.1126/science.aap8062
13. Seismic fault detection in real data using transfer learning from a convolutional neural network pre-trained with synthetic seismic data / A. Cunha, A. Pochet, H. Lopes, M. Gattass // Computers & Geosciences. – 2019. – Vol. 135. – DOI: 10.1016/j.cageo.2019.104344
14. Koroteev D., Tekic Z. Artificial intelligence in oil and gas upstream: Trends, challenges, and scenarios for the future // Energy and AI. – 2021. – Vol. 3. – DOI: 10.1016/j.egyai.2020.100041
15. Applications of artificial intelligence in oil and gas development / Hong Li, Haiyang Yu, Nai Cao [et al.] // Archives of Computational Methods in Engineering. – 2020. – Vol. 28, Issue 3. – P. 937–949. – DOI: 10.1007/s11831-020-09402-8
16. Subsurface velocity inversion from deep learning-based data assimilation / Bo Mao, Li-Guo Han, Qiang Feng, Yu-Chen Yin // J. of Applied Geophysics. – 2019. – Vol. 167. – P. 172–179. – DOI: 10.1016/j.jappgeo.2019.04.002
17. Decision support methods and applications in the upstream oil and gas sector / M. Shafiee, I. Animah, B. Alkali, D. Baglee // J. of Petroleum Science and Engineering. – 2019. – Vol. 173. – P. 1173–1186. – DOI: 10.1016/j.petrol.2018.10.050
18. Strantzali E., Aravossis K. Decision making in renewable energy investments: a review // Renewable and Sustainable Energy Reviews. – 2016. – Vol. 55. – P. 885–898. – DOI: 10.1016/j.rser.2015.11.021