Scientific and economic journal
«Problems of economics and management of oil and gas complex»
ISSN 1999-6942
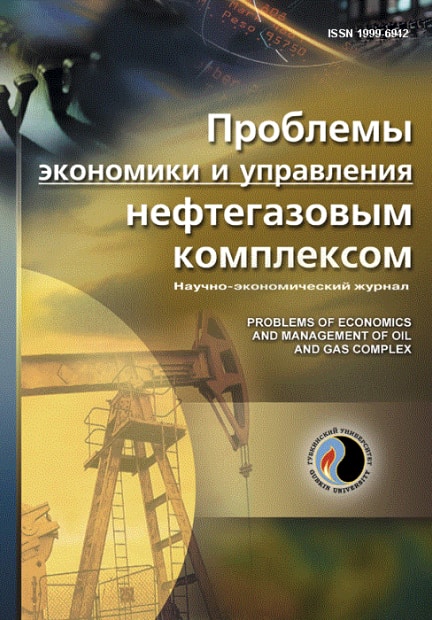
Studying the directions of the oil and gas complex development through the prism of "learning processes"
UDC: 330.3+338.2
DOI: -
Authors:


1 Institute of Economics and Industrial Engineering, Siberian Branch of the Russian Academy of Sciences, Novosibirsk, Russia
2 National Research University Higher School of Economics, Moscow, Russia
Keywords: oil and gas complex, machine learning, artificial intelligence, innovation, economic frameworks, knowledge transfer, government agencies & institutions
Annotation:
In the oil and gas industry, data processing has always played and will continue to play a key role. However, their structure and quality change significantly over time. The importance of data that characterize technical and related management decision-making and implementation, reflecting institutional conditions and frameworks in different countries and activity spheres, is increasing rapidly. Due to the complexity of the real experiment on the institutional conditions influence on the process of certain decisions implementation, it is possible to apply approaches on the basis of "learning processes" – first on the basis of qualitative studying the directions of the studied objects development, and then machine learning models (MLM) and artificial intelligence (AI). In the authors opinion, the success of the application of new technological and organizational-economic approaches in the oil service sector is largely related to the development of scientific research, first of all of a complex nature; i.e., close interaction of both processes of new technologies development and determination of framework conditions of their effective application.
Bibliography:
1. Boschma R. Relatedness as driver of regional diversification: a research agenda // Regional Studies. – 2017. – Vol. 51, Issue 3. – P. 351–364. – DOI: 10.1080/00343404.2016.12547672. Long R. DOE’s Unconventional Gas Research Programs 1976–1995: An Archive of Important Results. – National Energy Technology Laboratory, U.S. Department of Energy, 2016. – URL: https://edx.netl.doe.gov/dataset/doe-s-unconventional-gas-research-programs-1976-1995 (data obrashcheniya 15.12.2023).
3. Burwen J., Flegal J. Unconventional Gas Exploration & Production. – American Energy Innovation Council, 2013. – 16 p. – URL: https://bipartisanpolicy.org/download/?file=/wp-content/uploads/2013/03/Case-Unconventional-Gas.pdf
4. Covert T.R. Experiential and Social Learning in Firms: The Case of Hydraulic Fracturing in the Bakken Shale. – 2015. – 50 p. – DOI: 10.2139/SSRN.2481321
5. Liu Z., Schiff A., Swem N. Access to Capital and Investment Composition: Evidence from Fracking in North Dakota. – 2018. – 72 p. – DOI: 10.2139/SSRN.3224515
6. A Comprehensive Review of Smart/Intelligent Oilfield Technologies and Applications in the Oil and Gas Industry / C. Temizal, C.H. Canbaz, Y. Palabiyik [et al.] // SPE Middle East Oil and Gas Show and Conf., Manama, Bahrain, March 18–21, 2019. – DOI: 10.2118/195095-MS
7. Pandey R.K., Dahiya A.K., Mandal A. Identifying Applications of Machine Learning and Data Analytics Based Approaches for Optimization of Upstream Petroleum Operations // Energy Technology. – 2021. – Vol. 9, Issue 1. – DOI: 10.1002/ENTE.202000749
8. Roy D.G., Singh T.N., Kodikara J. Predicting mode-I fracture toughness of rocks using soft computing and multiple regression // Measurement. – 2018. – Vol. 126. – P. 231–241. – DOI: 10.1016/J.MEASUREMENT.2018.05.069
9. New Model for Pore Pressure Prediction While Drilling Using Artificial Neural Networks / A. Ahmed, S. Elkatatny, A. Ali [et al.] // Arabian J. for Science and Engineering. – 2019. – Vol. 44. – P. 6079–6088. – DOI: 10.1007/S13369-018-3574-7
10. Malallah A., Nashawi I.S. Estimating the fracture gradient coefficient using neural networks for a field in the Middle East // J. of Petroleum Science and Engineering. – 2005. – Vol. 49, Issue 3–4. – P. 193–211. – DOI: 10.1016/J.PETROL.2005.05.006
11. Li X., Chan C.W., Nguyen H.H. Application of the Neural Decision Tree Approach for Prediction of Petroleum Production // J. of Petroleum Science and Engineering. – 2013. – Vol. 104. – P. 11–16. – DOI: 10.1016/J.PETROL.2013.03.018
12. Xinli Jia, Feifei Zhang. Applying Data-Driven Method to Production Decline Analysis and Forecasting // SPE Annual Technical Conf. and Exhibition, Dubai, UAE, Sept. 26–28, 2016. – DOI: 10.2118/181616-MS
13. Application of artificial intelligence to forecast hydrocarbon production from shales / P. Panja, R. Velasco, M. Pathak, M. Deo // Petroleum. – 2018. – Vol. 4, Issue 1. – P. 75–89. – DOI: 10.1016/J.PETLM.2017.11.003
14. Shokir E.M.El-M. A Novel Model for Permeability Prediction in Uncored Wells // SPE Reservoir Evaluation & Engineering. – 2006. – Vol. 9, Issue 3. – P. 266–273. – DOI: 10.2118/87038-PA
15. Kenari S.A.J., Mashohor S. Robust committee machine for water saturation prediction // J. of Petroleum Science and Engineering. – 2013. – Vol. 104. – P. 1–10. – DOI: 10.1016/J.PETROL.2013.03.009
16. Experimental study and artificial neural network simulation of the wettability of tight gas sandstone formation / Yayun Zhang, Mian Chen, Yan Jin [et al.] // J. of Natural Gas Science and Engineering. – 2016. – Vol. 34. – P. 387–400. – DOI: 10.1016/J.JNGSE.2016.07.002
17. An Integrated Closed-loop Solution to Assisted History Matching and Field Optimization with Machine Learning Techniques / Zhi Chai, A. Nwachukwu, Y. Zagayevskiy [et al.] // J. of Petroleum Science and Engineering. – Vol. 198. – DOI: 10.1016/J.PETROL.2020.108204
18. Shafranik Yu.K., Kryukov V.A. Neftegazovyy sektor Rossii: trudnyy put' k mnogoobraziyu. – M.: Pero, 2016. – 271 s.
19. Razmanova S.V., Andrukhova O.V. Oilfield service companies as part of economy digitalization: assessment of the prospects for innovative development // J. of Mining Institute. – 2020. – Vol. 244. – P. 482–492. – DOI:10.31897/PMI.2020.4.11
20. Luki N.B. Are There Good Institutions in Ghana’s Oil and Gas Industry? // Developing Country Studies. – 2016. – Vol. 6, Issue 9. – P. 23–56.
21. Kryukov V.A. Ob institutsionalizatsii roli i mesta krupnogo biznesa v reshenii problem sotsial'no-ekonomicheskogo razvitiya strany // Ekonomicheskoe vozrozhdenie Rossii. – 2023. – № 2(76). – S. 42–52. – DOI: 10.37930/1990-9780-2023- 2(76)-42-52