Scientific and technical journal
«Proceedings of Gubkin University»
ISSN 2073-9028
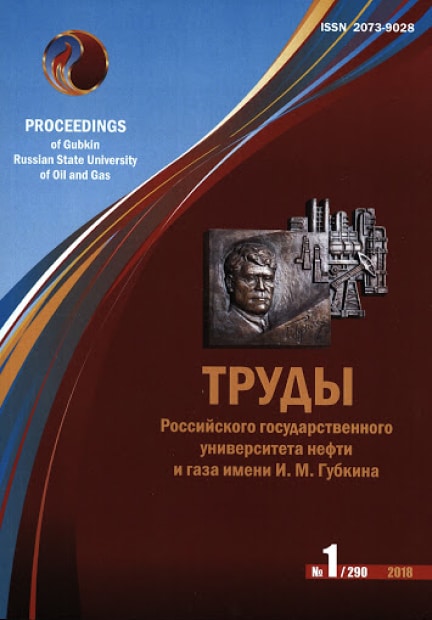
Revisiting the issue of preparation of initial array of information for training neural networks to determine parameters of pipeline defects
UDC: 620.19:621.43
DOI: 10.33285/2073-9028-2023-2(311)-85-97
Authors:



1 Gazprom Diagnostics, St. Petersburg, Russian Federation
2 National University of Oil and Gas “Gubkin University”, Moscow, Russian Federation
Keywords: defects, in-line diagnostics, neural network
Annotation:
The article presents preliminary work and analysis of historical data on identified defects by the method of in-line diagnostics (ITD) for subsequent training of the neural network. The analysis focuses on the repeatability of results from the reports of different years and on the convergence of the data, taking into account the same operating conditions on adjacent pipelines. The sample size for analysis was more than 15,5 million defects with different sizes and characteristics. It is shown that repeated examinations of the VTD make it possible to assess the technical condition of pipelines, identify the causes of the formation and growth dynamics of the detected defects, identify the areas of high corrosive activity, and also form reasonable proposals for the diagnostics, maintenance and repair plan.
Bibliography:
1. Skrynnikov S.V. Sovremennye principy i napravlenija razvitija sistemy organizacii diagnostiki, tehnicheskogo obsluzhivanija i remonta v PAO “Gazprom”//Gazovaja promyshlennost’. – 2017. – № S2 (754). – S. 4–9.2. Moubray J. Reliability-centered Maintenance. – NY: Industrial Press Inc., 2001. – 426 р.
3. Olejnikov A.V., Kazak A.S. Sovremennoe sostojanie i perspektivy cifrovoj transformacii v neftegazovyh kompanijah//Avtomatizacija i informatizacija TJeK. – 2023. – № 3 (596). – S. 12–27. – DOI: 10.33285/2782-604X-2023-3(596)-12-27
4. Anifowose F., Labadin J., Abdulraheem A. Ensemble machine learning: an untapped modeling paradigm for petroleum reservoir characterization//Journal of Petroleum Science and Engineering. – 2017. – Vol. 151. – P. 480–487. – DOI: 10.1016/j.petrol.2017.01.024
5. Improved recurrent neural network-based manipulator control with remote center of motion constraints: Experimental results’/Su Hang, Hu Yingbai, H.R. Karimi [et al.]//Neural Netw. –November. – 2020. – DOI: 10.1016/j.neunet.2020.07.033
6. Sovremennye instrumenty biznes-analitiki kak kljuch dlja povyshenija jeffektivnosti raboty informacionnoj sistemy/A.V. Shibanov, D.V. Tre’jakov, K.V. Ovodkova, K.N. Zhuchkov//Avtomatizacija i informatizacija TJeK. – 2023. – № 1 (594). – S. 5–11. – DOI: 10.33285/2782-604X-2023-1(594)-5-11
7. Zhuchkov K.N., Zavyalov A.P. Sovershenstvovanie tehnologii vnutritrubnoj diagnostiki truboprovodov s ispol’zovaniem algoritma avtomatizirovannoj obrabotki diagnosticheskih dannyh//Nauka i tehnologii truboprovodnogo transporta nefti i nefteproduktov. – 2022. – № 12 (6). – S. 540–549. – DOI: 10.28999/2541-9595-2022-12-6-540-549
8. Acoustic detection and localization of gas pipeline leak based on residual connection and one-dimensional separable convolutional neural network/W. Yan, W. Liu, H. Bi [et al.]//Transactions of the Institute of Measurement and Control. – 2023. – DOI:10.1177/01423312231156264
9. Diagnosis and Recognition of Pipeline Damage Defects Based on Neural Network Algorithm/M. Zhang, Y. Guo, D. Wang, Q. Du//Proceedings of the 2022 14th International Pipeline Conference. Volume 1: Pipeline Safety Management Systems; Project Management, Design, Construction, and Environmental Issues; Strain-Based Design and Assessment; Risk and Reliability; Emerging Fuels and Greenhouse Gas Emissions. – Calgary, Alberta, Canada. September 26–30, 2022. V001T01A013. ASME. – URL: https://doi.org/10.1115/IPC2022-87163
10. Zavyalov A., Zhuchkov K., Vasilchenko M. Process Pipeline Strength Calculation Methodology Enhancement Using Finite-Element Method//Journal of Pipeline Systems Engineering and Practice. – 2023. – Vol. 14, № 2. – DOI: 10.1061/JPSEA2.PSENG-1401
11. Improving the accuracy of estimates of the pulse sequence period using the methodology of complete sufficient statistics/K. Zhuchkov, M. Vasilchenko, A. Zagrebneva, A. Zavyalov//Scientific Reports. – 2022. – Vol. 12, № 1. – P. 19932. – DOI: 10.1038/s41598-022-24457-2
12. Analiticheskie i tehnicheskie aspekty diagnostiki linejnoj chasti gazoprovodov/I.I. Velijulin, V.I. Gorodnichenko, V.A. Aleksandrov [i dr.]//Territorija Neftegaz. – 2021. – № 7–8. – S. 44–55. – EDN BALTXY.
13. Vasilchenko M., Zavyalov A., Zhuchkov K. Increasing the Stability of a Spatially Distributed Information System Using a Robust Algorithm for Filtering Anomalous Measurements//Inf. Technol. Ind. – 2020. – Vol. 8, № 3. – P. 1–7.
14. Issledovanie osobennostej KRN magistral’nyh gazoprovodov bol’shogo diametra/V.A. Seredenok, V.L. Onackij, V.N. Tolkacheva, R.V. Aginej//Truboprovodnyj transport: teorija i praktika. – 2016. – № 5 (57). – S. 12–16. – EDN WYPNHD.