Scientific and technical journal
«Environmental protection in oil and gas complex»
ISSN 2411-7013
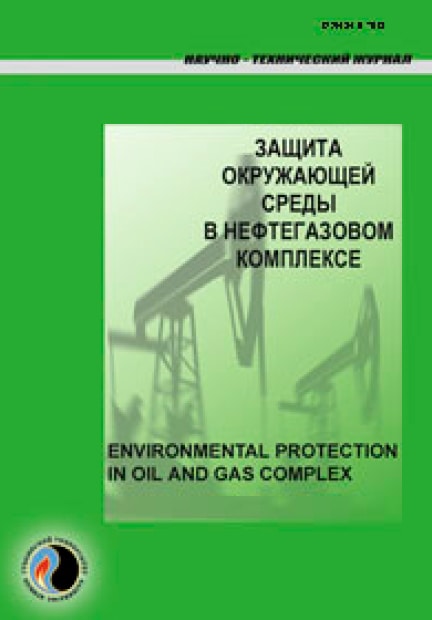
Geological-statistical logical-informational model for assessing the impact of liquid oil-containing waste on the geological environment
UDC: 502.521
DOI: -
Authors:




1 National University of Oil and Gas "Gubkin University", Moscow, Russia
2 National Association for the Prevention and Response to Oil Spills, Moscow, Russia
Keywords: oil-containing waste, ecological anomaly, integration of methods, logical-information model, geological environment, monitoring, geoctatistical analysis
Annotation:
The methodology for monitoring studies of ecological anomalies is proposed, which takes into account the observation network structuring, the accuracy of measurements, the principles of processing, as well as the interpretation of the data obtained. When developing a methodology, a key approach is the geostatistical analysis of the impact aftereffects on the environment of liquid oily wastes. An adaptable logical-informational model is proposed for effective integration of environmental monitoring methods and appropriate forecasting. The logical-informational model takes into account the network of observations, the accuracy of measurements, the principles of processing and interpreting the data obtained using the Morishita diagram. The analysis of geospatial information makes it possible to predictively model the spread of the most common pollutants and visualize their results and develop recommendations for their elimination. The results of a geological-statistical analysis of the aftereffects of liquid oily waste impact on the geological environment were used to form an observation network and interpret monitoring data.
Bibliography:
1. Ostakh S.V., Ostakh O.S., Ol'khovikova N.Yu. Kontseptsiya sozdaniya eshelonirovannoy sistemy zashchity prirodno-antropogennykh kompleksov // Ekologiya i prom-st' Rossii. – 2019. – T. 23, № 3. – S. 54–59. – DOI: 10.18412/1816-0395-2019-3-54-592. Dimakova N.A., Sharapov R.V. Problema zagryazneniya podzemnykh vod // Sovremennye naukoemkie tekhnologii. – 2013. – № 2. – S. 79–82.
3. Khelyal' M.A. Vozmozhnosti geofizicheskikh metodov pri ekologicheskikh issledovaniyakh // Sovremennye problemy inzhenernykh izyskaniy na territorii Tsentral'no-Chernozemnogo regiona: materialy 1-y region. nauch.-prakt. konf., Voronezh, 10–11 fevr. 2017 g. – Voronezh: Nauch. kn., 2017. – S. 177–181.
4. Kompleksirovanie metodov gidrogeologicheskogo i gidroekologicheskogo prognozirovaniya posledstviy vozdeystviya na litosferu nesanktsionirovano razmeshchennykh otkhodov / S.V. Ostakh, M.P. Papini, P. Chiampi, N.Yu. Ol'khovikova // Zashchita okruzhayushchey sredy v neftegazovom komplekse. – 2020. – № 5(296). – S. 12–20. – DOI: 10.33285/2411-7013-2020-5(296)-12-20
5. Divina T.V., Petrakova E.A., Vishnevskiy M.S. Osnovnye metody analiza ekspertnykh otsenok // Ekonomika i biznes: teoriya i praktika. – 2019. – № 7. – S. 42–44. – DOI: 10.24411/2411-0450-2019-11072
6. Kochumeev V.A., Mirmanov A.B., Stukach O.V. Izuchenie problemnykh situatsiy v razrabotke perspektivnykh geofizicheskikh informatsionno-izmeritel'nykh sistem // Vestn. nauki Sibiri. – 2012. – № 3(4). – S. 99–102.
7. Borovskiy M.Ya., Shakuro S.V., Bogatov V.I. Geofizicheskaya razvedka tekhnogennykh istochnikov uglevodorodnogo syr'ya // Voprosy teorii i praktiki geologicheskoy interpretatsii geofizicheskikh poley: materialy 43-y ses. Mezhdunar. nauch. seminara im. D.G. Uspenskogo, Voronezh, 26–30 yanv. 2016 g. – Voronezh: Nauch. kn., 2016. – S. 35–39.
8. Avsent'ev A.O. Opredelenie tsennosti informatsii // Dokl. Tomskogo gos. un-ta sistem upravleniya i radioelektroniki. – 2016. – T. 19, № 1. – S. 21–24. – DOI: 10.21293/1818-0442-2016-19-1-21-24
9. Minnimukhametova A.A. Geoinformatsionnoe kartografirovanie // Simvol nauki: mezhdunar. nauch. zhurn. – 2016. – № 8-1(20). – S. 24–25.
10. Morisita M. Measuring the dispersion of individuals and analysis of the distributional patterns // Memories of the Faculty of Science, Kyushu University. Series E: Biology. – 1959. – Vol. 2. – P. 215–235.
11. The Multipoint Morisita Index for the Analysis of Spatial Patterns / J. Golay, M. Kanevski, C.D. Vega Orozco, M. Leuenberger // Physica A: Statistical Mechanics and Its Applications. – 2014. – Vol. 406. – P. 191–202. – DOI: 10.1016/j.physa.2014.03.063
12. Cross-validation strategies for data with temporal, spatial, hierarchical, or phylogenetic structure / D.R. Roberts, V. Bahn, S. Ciuti [et al.] // Ecography. – 2017. – Vol. 40, Issue 8. – P. 913–929. – DOI: 10.1111/ecog.02881
13. Shitikov V.K., Mastitskiy S.E. Klassifikatsiya, regressiya i drugie algoritmy Data Mining s ispol'zovaniem R. – Tol'yatti; London, 2017. – 351 s. – URL: https://github.com/ranalytics/data-mining (data obrashcheniya 25.11.2023).
14. Murty M.N., Devi V.S. Introduction to pattern recognition and machine learning. – World Scientific Publishing Co. Pte. Ltd., 2015. – 402 p.
15. Bayesian leave-oneout cross-validation approximations for Gaussian latent variable models / A. Vehtari, T. Mononen, V. Tolvanen [et al.] // J. of Machine Learning Research. – 2016. – Vol. 17. – Article No. 103. – P. 1–38. – DOI: 10.48550/arXiv.1412.7461